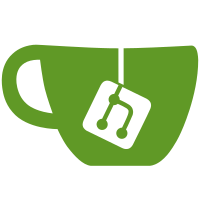
7 changed files with 775 additions and 0 deletions
@ -0,0 +1,8 @@ |
|||
# 默认忽略的文件 |
|||
/shelf/ |
|||
/workspace.xml |
|||
# 基于编辑器的 HTTP 客户端请求 |
|||
/httpRequests/ |
|||
# Datasource local storage ignored files |
|||
/dataSources/ |
|||
/dataSources.local.xml |
@ -0,0 +1,41 @@ |
|||
import math |
|||
|
|||
class Config: |
|||
def __init__(self): |
|||
# 目录提取拼接相关参数 |
|||
self.pantten_second_biaoti = '[2二ⅡⅠ][、.]\s{0,}?[\u4e00-\u9fa5]+' |
|||
self.pantten_other_biaoti = '[2-9二三四五六七八九ⅡⅢⅣⅤⅥⅦⅧⅨ][、.]\s{0,}?[\u4e00-\u9fa5]+' |
|||
self.pantten_biaoti = '[1-9一二三四五六七八九ⅠⅡⅢⅣⅤⅥⅦⅧⅨ][、.]\s{0,}?[\u4e00-\u9fa5a-zA-Z]+' |
|||
|
|||
# chatgpt 接口相关参数 |
|||
|
|||
self.mulu_prompt = "生成目录#\n问:为论文题目《{}》生成目录,要求只有一级标题和二级标题,一级标题使用中文数字 例如一、xxx;二级标题使用阿拉伯数字 例如1.1 xxx;一级标题不少于7个;每个一级标题至少包含3个二级标题\n答:\n" |
|||
self.first_title_prompt = "生成论文小标题内容#\n问:论文题目是《{}》,目录是“{}”,请把其中的大标题“{}”的内容补充完整,补充内容字数在{}字左右\n答:\n" |
|||
self.small_title_prompt = "生成论文小标题内容#\n问:论文题目是《{}》,目录是“{}”,请把其中的小标题“{}”的内容补充完整,补充内容字数在{}字左右\n答:\n" |
|||
self.references_prompt = "论文题目是“{}”,目录是“{}”,请为这篇论文生成15篇左右的参考文献,要求其中有有中文参考文献不低于12篇,英文参考文献不低于2篇" |
|||
self.thank_prompt = "请以“{}”为题写一篇论文的致谢" |
|||
self.kaitibaogao_prompt = "请以《{}》为题目生成研究的主要的内容、背景、目的、意义,要求不少于1500字" |
|||
self.chinese_abstract_prompt = "生成论文摘要#\n问:论文题目是《{}》,目录是“{}”,生成论文摘要,要求生成的字数在600字左右\n答:\n" |
|||
self.english_abstract_prompt = "翻译摘要#\n问:请把“{}”这段文字翻译成英文\n答:\n" |
|||
self.chinese_keyword_prompt = "生成关键字#\n问:请为“{}”这段论文摘要生成3-5个关键字,使用阿拉伯数字作为序号标注,例如“1.xxx \n2.xxx \n3.xxx \n4.xxx \n5.xxx \n”\"\n答:\n" |
|||
self.english_keyword_prompt = "翻译关键词#\n问:请把“{}”这几个关键字翻译成英文\n答:\n" |
|||
self.dabiaoti = ["二", "三", "四", "五", "六", "七", "八", "九"] |
|||
self.project_data_txt_path = "/home/majiahui/project2/LLaMA-Efficient-Tuning/new_data_txt_4" |
|||
|
|||
# 流程相关参数 |
|||
self.thanks = "致谢" |
|||
self.references = "参考文献" |
|||
self.excursus = "附录" |
|||
self.u = 3.5 # 均值μ |
|||
self.sig = math.sqrt(6.0) |
|||
self.zong_gradient = 6 |
|||
self.paper_word_count = 12000 |
|||
|
|||
# flask port |
|||
self.flask_port = "14003" |
|||
|
|||
# redis config |
|||
self.reids_ip = '192.168.31.145' |
|||
self.reids_port = 6379 |
|||
self.reids_db = 7 |
|||
self.reids_password = 'Zhicheng123*' |
@ -0,0 +1,61 @@ |
|||
from flask import Flask, jsonify |
|||
from flask import request |
|||
from transformers import pipeline |
|||
import redis |
|||
import uuid |
|||
import json |
|||
from threading import Thread |
|||
from vllm import LLM, SamplingParams |
|||
import time |
|||
|
|||
app = Flask(__name__) |
|||
app.config["JSON_AS_ASCII"] = False |
|||
pool = redis.ConnectionPool(host='localhost', port=63179, max_connections=50,db=11, password="zhicheng123*") |
|||
redis_ = redis.Redis(connection_pool=pool, decode_responses=True) |
|||
|
|||
db_key_query = 'query' |
|||
db_key_result = 'result' |
|||
batch_size = 32 |
|||
|
|||
sampling_params = SamplingParams(temperature=0.95, top_p=0.7,presence_penalty=0.9,stop="</s>", max_tokens=2048) |
|||
models_path = "/home/majiahui/project/models-llm/openbuddy-llama-7b-finetune" |
|||
llm = LLM(model=models_path, tokenizer_mode="slow") |
|||
|
|||
def classify(batch_size): # 调用模型,设置最大batch_size |
|||
while True: |
|||
texts = [] |
|||
query_ids = [] |
|||
if redis_.llen(db_key_query) == 0: # 若队列中没有元素就继续获取 |
|||
time.sleep(2) |
|||
continue |
|||
for i in range(min(redis_.llen(db_key_query), batch_size)): |
|||
query = redis_.lpop(db_key_query).decode('UTF-8') # 获取query的text |
|||
query_ids.append(json.loads(query)['id']) |
|||
texts.append(json.loads(query)['text']) # 拼接若干text 为batch |
|||
outputs = llm.generate(texts, sampling_params) # 调用模型 |
|||
for (id_, output) in zip(query_ids, outputs): |
|||
res = output.outputs[0].text |
|||
redis_.set(id_, json.dumps(res)) # 将模型结果送回队列 |
|||
|
|||
|
|||
@app.route("/predict", methods=["POST"]) |
|||
def handle_query(): |
|||
text = request.json["texts"] # 获取用户query中的文本 例如"I love you" |
|||
id_ = str(uuid.uuid1()) # 为query生成唯一标识 |
|||
d = {'id': id_, 'text': text} # 绑定文本和query id |
|||
redis_.rpush(db_key_query, json.dumps(d)) # 加入redis |
|||
while True: |
|||
result = redis_.get(id_) # 获取该query的模型结果 |
|||
if result is not None: |
|||
redis_.delete(id_) |
|||
result_text = {'code': "200", 'data': json.loads(result)} |
|||
break |
|||
time.sleep(1) |
|||
|
|||
return jsonify(result_text) # 返回结果 |
|||
|
|||
|
|||
if __name__ == "__main__": |
|||
t = Thread(target=classify, args=(batch_size,)) |
|||
t.start() |
|||
app.run(debug=False, host='0.0.0.0', port=9000) |
@ -0,0 +1,43 @@ |
|||
import time |
|||
from flask import Flask, jsonify |
|||
from flask import request |
|||
from vllm import LLM, SamplingParams |
|||
|
|||
app = Flask(__name__) |
|||
app.config["JSON_AS_ASCII"] = False |
|||
# prompts = [ |
|||
# "生成论文小标题内容#问:论文题目是“大学生村官管理研究”,目录是“一、大学生村官管理现状分析\\n1.1 村官数量及分布情况\\n1.2 村官岗位设置及职责\\n1.3 村官工作绩效评估\\n\\n二、大学生村官管理存在的问题\\n2.1 村官队伍结构不合理\\n2.2 村官工作能力不足\\n2.3 村官管理制度不健全\\n\\n三、大学生村官管理对策研究\\n3.1 加强村官队伍建设\\n3.2 提高村官工作能力\\n3.3 完善村官管理制度\\n\\n四、大学生村官管理案例分析\\n4.1 案例一:某村大学生村官工作情况分析\\n4.2 案例二:某村大学生村官管理策略探讨\\n\\n五、大学生村官管理的未来发展趋势\\n5.1 多元化村官队伍建设\\n5.2 信息化村官管理模式\\n5.3 村官职业化发展\\n\\n六、大学生村官管理的政策建议\\n6.1 加强对大学生村官的培训和管理\\n6.2 完善大学生村官管理制度\\n6.3 提高大学生村官的待遇和福利\\n\\n七、结论与展望”,请把其中的小标题“3.3 完善村官管理制度”的内容补充完整,补充内容字数在800字左右\n答:\n" |
|||
# ] * 10 |
|||
sampling_params = SamplingParams(temperature=0.95, top_p=0.7,presence_penalty=0.9,stop="</s>") |
|||
# |
|||
model_path = '/home/majiahui/project/models-llm/openbuddy-llama-7b-finetune' |
|||
llm = LLM(model=model_path) |
|||
# |
|||
# t1 = time.time() |
|||
# outputs = llm.generate(prompts, sampling_params) |
|||
|
|||
|
|||
@app.route("/predict", methods=["POST"]) |
|||
def handle_query(): |
|||
print(request.remote_addr) |
|||
texts = request.json["texts"] |
|||
print(type(texts)) |
|||
outputs = llm.generate(texts, sampling_params) |
|||
print(outputs) |
|||
print("===========================================================================================================") |
|||
# generated_text = outputs[0].outputs[0].text |
|||
print(len(texts)) |
|||
generated_text_list = [""] * len(texts) |
|||
for i,output in enumerate(outputs): |
|||
index = output.request_id |
|||
print(index) |
|||
try: |
|||
generated_text = output.outputs[0].text |
|||
generated_text_list[int(index)] = generated_text |
|||
except: |
|||
print(output) |
|||
result_text = {'code': "200", 'data': generated_text_list} |
|||
return jsonify(result_text) # 返回结果 |
|||
|
|||
if __name__ == "__main__": |
|||
app.run(host="0.0.0.0", port=15001, threaded=True, debug=False) |
@ -0,0 +1,481 @@ |
|||
import os |
|||
|
|||
os.environ["CUDA_VISIBLE_DEVICES"] = "0" |
|||
from flask import Flask, jsonify |
|||
from flask import request |
|||
# from linshi import autotitle |
|||
import requests |
|||
import redis |
|||
import uuid |
|||
import json |
|||
from threading import Thread |
|||
import time |
|||
import re |
|||
import logging |
|||
from config_llama_api import Config |
|||
import numpy as np |
|||
import math |
|||
from transformers import AutoModelForCausalLM, AutoTokenizer, TextStreamer |
|||
import torch |
|||
from vllm import LLM, SamplingParams |
|||
|
|||
config = Config() |
|||
|
|||
model_path = '/home/majiahui/models-LLM/openbuddy-llama-7b-finetune-v3' |
|||
# model_path = '/home/majiahui/models-LLM/openbuddy-openllama-7b-v5-fp16' |
|||
# model_path = '/home/majiahui/models-LLM/baichuan-vicuna-chinese-7b' |
|||
# model_path = '/home/majiahui/models-LLM/openbuddy-llama-7b-v1.4-fp16' |
|||
|
|||
sampling_params = SamplingParams(temperature=0.95, top_p=0.7,presence_penalty=0.9,stop="</s>") |
|||
models_path = model_path |
|||
llm = LLM(model=models_path) |
|||
|
|||
|
|||
# WEIGHTS_NAME = "adapter_model.bin" |
|||
# checkpoint_dir = "/home/majiahui/project2/LLaMA-Efficient-Tuning/path_to_sft_checkpoint_paper_prompt_freeze_checkpoint_new_48000/checkpoint-16000" |
|||
# weights_file = os.path.join(checkpoint_dir, WEIGHTS_NAME) |
|||
# assert os.path.exists(weights_file), f"Provided path ({checkpoint_dir}) does not contain the pretrained weights." |
|||
# model_state_dict = torch.load(weights_file, map_location="cuda") |
|||
# model.load_state_dict(model_state_dict, strict=False) # skip missing keys |
|||
# model = model.cuda() |
|||
|
|||
redis_title = "redis_title" |
|||
pool = redis.ConnectionPool(host=config.reids_ip, port=config.reids_port, max_connections=50, db=config.reids_db) |
|||
redis_ = redis.Redis(connection_pool=pool, decode_responses=True) |
|||
|
|||
app = Flask(__name__) |
|||
app.config["JSON_AS_ASCII"] = False |
|||
|
|||
# mulu_prompt = "为论文题目“{}”生成目录,要求只有一级标题和二级标题,一级标题使用中文数字 例如一、xxx;二级标题使用阿拉伯数字 例如1.1 xxx;一级标题不少于7个;每个一级标题至少包含3个二级标题" |
|||
# first_title_prompt = "论文题目是“{}”,目录是“{}”,请把其中的大标题“{}”的内容补充完整,补充内容字数在{}字左右" |
|||
# small_title_prompt = "论文题目是“{}”,目录是“{}”,请把其中的小标题“{}”的内容补充完整,补充内容字数在{}字左右" |
|||
# references_prompt = "论文题目是“{}”,目录是“{}”,请为这篇论文生成15篇左右的参考文献,要求其中有有中文参考文献不低于12篇,英文参考文献不低于2篇" |
|||
# thank_prompt = "请以“{}”为题写一篇论文的致谢" |
|||
# kaitibaogao_prompt = "请以《{}》为题目生成研究的主要的内容、背景、目的、意义,要求不少于1500字" |
|||
# chinese_abstract_prompt = "请以《{}》为题目生成论文摘要,要求生成的字数在600字左右" |
|||
# english_abstract_prompt = "请把“{}”这段文字翻译成英文" |
|||
# chinese_keyword_prompt = "请为“{}”这段论文摘要生成3-5个关键字,使用阿拉伯数字作为序号标注,例如“1.xxx \\n2.xxx \\n3.xxx \\n4.xxx \\n5.xxx \\n" |
|||
# english_keyword_prompt = "请把“{}”这几个关键字翻译成英文" |
|||
|
|||
|
|||
def normal_distribution(x): |
|||
y = np.exp(-(x - config.u) ** 2 / (2 * config.sig ** 2)) / (math.sqrt(2 * math.pi) * config.sig) |
|||
return y |
|||
|
|||
|
|||
def request_chatglm(prompt): |
|||
outputs = llm.generate([prompt], sampling_params) |
|||
generated_text = outputs[0].outputs[0].text |
|||
return generated_text |
|||
|
|||
|
|||
def chat_kaitibaogao(main_parameter): |
|||
response = request_chatglm(config.kaitibaogao_prompt.format(main_parameter[0])) |
|||
|
|||
return response |
|||
|
|||
|
|||
def chat_abstract_keyword(main_parameter): |
|||
# 生成中文摘要 |
|||
|
|||
chinese_abstract = request_chatglm(config.chinese_abstract_prompt.format(main_parameter[0],main_parameter[1])) |
|||
|
|||
# 生成英文的摘要 |
|||
|
|||
english_abstract = request_chatglm(config.english_abstract_prompt.format(chinese_abstract)) |
|||
|
|||
# 生成中文关键字 |
|||
|
|||
chinese_keyword = request_chatglm(config.chinese_keyword_prompt.format(chinese_abstract)) |
|||
|
|||
# 生成英文关键字 |
|||
english_keyword = request_chatglm(config.english_keyword_prompt.format(chinese_keyword)) |
|||
|
|||
paper_abstract_keyword = { |
|||
"中文摘要": chinese_abstract, |
|||
"英文摘要": english_abstract, |
|||
"中文关键词": chinese_keyword, |
|||
"英文关键词": english_keyword |
|||
} |
|||
|
|||
return paper_abstract_keyword |
|||
|
|||
|
|||
def chat_content(main_parameter): |
|||
''' |
|||
|
|||
:param api_key: |
|||
:param uuid: |
|||
:param main_parameter: |
|||
:return: |
|||
''' |
|||
content_index = main_parameter[0] |
|||
title = main_parameter[1] |
|||
mulu = main_parameter[2] |
|||
subtitle = main_parameter[3] |
|||
prompt = main_parameter[4] |
|||
word_count = main_parameter[5] |
|||
|
|||
if subtitle[:2] == "@@": |
|||
response = subtitle[2:] |
|||
else: |
|||
response = request_chatglm(prompt.format(title, mulu, subtitle, word_count)) |
|||
if subtitle not in response: |
|||
response = subtitle + "\n" + response |
|||
|
|||
print(prompt.format(title, mulu, subtitle, word_count), response) |
|||
return response |
|||
|
|||
|
|||
def chat_thanks(main_parameter): |
|||
''' |
|||
|
|||
:param api_key: |
|||
:param uuid: |
|||
:param main_parameter: |
|||
:return: |
|||
''' |
|||
# title, |
|||
# thank_prompt |
|||
title = main_parameter[0] |
|||
prompt = main_parameter[1] |
|||
|
|||
response = request_chatglm(prompt.format(title)) |
|||
|
|||
return response |
|||
|
|||
|
|||
def chat_references(main_parameter): |
|||
''' |
|||
|
|||
:param api_key: |
|||
:param uuid: |
|||
:param main_parameter: |
|||
:return: |
|||
''' |
|||
# title, |
|||
# mulu, |
|||
# references_prompt |
|||
title = main_parameter[0] |
|||
mulu = main_parameter[1] |
|||
prompt = main_parameter[2] |
|||
|
|||
response = request_chatglm(prompt.format(title, mulu)) |
|||
|
|||
# 加锁 读取resis并存储结果 |
|||
|
|||
return response |
|||
|
|||
|
|||
def small_title_tesk(small_title): |
|||
''' |
|||
顺序读取子任务 |
|||
:return: |
|||
''' |
|||
task_type = small_title["task_type"] |
|||
main_parameter = small_title["main_parameter"] |
|||
|
|||
# "task_type": "paper_content", |
|||
# "uuid": uuid, |
|||
# "main_parameter": [ |
|||
# "task_type": "paper_content", |
|||
# "task_type": "chat_abstract", |
|||
# "task_type": "kaitibaogao", |
|||
|
|||
if task_type == "kaitibaogao": |
|||
# result = chat_kaitibaogao(main_parameter) |
|||
result = "" |
|||
|
|||
elif task_type == "chat_abstract": |
|||
result= chat_abstract_keyword(main_parameter) |
|||
|
|||
|
|||
elif task_type == "paper_content": |
|||
result = chat_content(main_parameter) |
|||
|
|||
elif task_type == "thanks_task": |
|||
# result = chat_thanks(main_parameter) |
|||
result = "" |
|||
|
|||
elif task_type == "references_task": |
|||
# result = chat_references(main_parameter) |
|||
result = "" |
|||
else: |
|||
result = "" |
|||
|
|||
print(result, task_type, main_parameter) |
|||
return result, task_type |
|||
|
|||
|
|||
def main_prrcess(title): |
|||
mulu = request_chatglm(config.mulu_prompt.format(title)) |
|||
mulu_list = mulu.split("\n") |
|||
mulu_list = [i.strip() for i in mulu_list if i != ""] |
|||
# mulu_str = "@".join(mulu_list) |
|||
|
|||
|
|||
mulu_list_bool = [] |
|||
for i in mulu_list: |
|||
result_biaoti_list = re.findall(config.pantten_biaoti, i) |
|||
if result_biaoti_list != []: |
|||
mulu_list_bool.append((i, "一级标题")) |
|||
else: |
|||
mulu_list_bool.append((i, "二级标题")) |
|||
|
|||
mulu_list_bool_part = mulu_list_bool[:3] |
|||
|
|||
if mulu_list_bool_part[0][1] != "一级标题": |
|||
redis_.lpush(redis_title, json.dumps({"id": uuid, "title": title}, ensure_ascii=False)) # 加入redis |
|||
redis_.persist(redis_title) |
|||
return |
|||
if mulu_list_bool_part[0][1] == mulu_list_bool_part[1][1] == mulu_list_bool_part[2][1] == "一级标题": |
|||
redis_.lpush(redis_title, json.dumps({"id": uuid, "title": title}, ensure_ascii=False)) # 加入redis |
|||
redis_.persist(redis_title) |
|||
return |
|||
|
|||
table_of_contents = [] |
|||
|
|||
thanks_references_bool_table = mulu_list_bool[-5:] |
|||
|
|||
# thanks = "致谢" |
|||
# references = "参考文献" |
|||
for i in thanks_references_bool_table: |
|||
if config.references in i[0]: |
|||
mulu_list_bool.remove(i) |
|||
if config.thanks in i[0]: |
|||
mulu_list_bool.remove(i) |
|||
if config.excursus in i[0]: |
|||
mulu_list_bool.remove(i) |
|||
|
|||
title_key = "" |
|||
# for i in mulu_list_bool: |
|||
# if i[1] == "一级标题": |
|||
# table_of_contents["@@" + i[0]] = [] |
|||
# title_key = "@@" + i[0] |
|||
# else: |
|||
# table_of_contents[title_key].append(i[0]) |
|||
|
|||
for i in mulu_list_bool: |
|||
if i[1] == "一级标题": |
|||
paper_dan = { |
|||
"title": "@@" + i[0], |
|||
"small_title": [], |
|||
"word_count": 0 |
|||
} |
|||
table_of_contents.append(paper_dan) |
|||
else: |
|||
table_of_contents[-1]["small_title"].append(i[0]) |
|||
|
|||
x_list = [0] |
|||
y_list = [normal_distribution(0)] |
|||
|
|||
gradient = config.zong_gradient / len(table_of_contents) |
|||
for i in range(len(table_of_contents) - 1): |
|||
x_gradient = x_list[-1] + gradient |
|||
x_list.append(x_gradient) |
|||
y_list.append(normal_distribution(x_list[-1])) |
|||
|
|||
dan_gradient = config.paper_word_count / sum(y_list) |
|||
|
|||
for i in range(len(y_list)): |
|||
table_of_contents[i]["word_count"] = dan_gradient * y_list[i] |
|||
|
|||
print(table_of_contents) |
|||
|
|||
print(len(table_of_contents)) |
|||
|
|||
table_of_contents_new = [] |
|||
for dabiaoti_index in range(len(table_of_contents)): |
|||
dabiaoti_dict = table_of_contents[dabiaoti_index] |
|||
table_of_contents_new.append([dabiaoti_dict["title"], 0]) |
|||
for xiaobiaoti in dabiaoti_dict["small_title"]: |
|||
table_of_contents_new.append( |
|||
[xiaobiaoti, int(dabiaoti_dict["word_count"] / len(dabiaoti_dict["small_title"]))]) |
|||
|
|||
small_task_list = [] |
|||
# api_key, |
|||
# index, |
|||
# title, |
|||
# mulu, |
|||
# subtitle, |
|||
# prompt |
|||
kaitibaogao_task = { |
|||
"task_type": "kaitibaogao", |
|||
"uuid": uuid, |
|||
"main_parameter": [title] |
|||
} |
|||
|
|||
chat_abstract_task = { |
|||
"task_type": "chat_abstract", |
|||
"uuid": uuid, |
|||
"main_parameter": [title, mulu] |
|||
} |
|||
small_task_list.append(kaitibaogao_task) |
|||
small_task_list.append(chat_abstract_task) |
|||
content_index = 0 |
|||
while True: |
|||
if content_index == len(table_of_contents_new): |
|||
break |
|||
subtitle, word_count = table_of_contents_new[content_index] |
|||
prompt = config.small_title_prompt |
|||
print(table_of_contents_new[1][0]) |
|||
if content_index == 0 and table_of_contents_new[1][0][:2] == "@@" and subtitle[:2] == "@@": |
|||
subtitle, prompt, word_count = subtitle[2:], config.first_title_prompt, 800 |
|||
|
|||
if content_index == len(table_of_contents_new) - 1 and subtitle[:2] == "@@": |
|||
subtitle, prompt, word_count = subtitle[2:], config.first_title_prompt, 800 |
|||
|
|||
print("请求的所有参数", |
|||
content_index, |
|||
title, |
|||
subtitle, |
|||
prompt, |
|||
word_count) |
|||
|
|||
paper_content = { |
|||
"task_type": "paper_content", |
|||
"uuid": uuid, |
|||
"main_parameter": [ |
|||
content_index, |
|||
title, |
|||
mulu, |
|||
subtitle, |
|||
prompt, |
|||
word_count |
|||
] |
|||
} |
|||
|
|||
small_task_list.append(paper_content) |
|||
content_index += 1 |
|||
|
|||
thanks_task = { |
|||
"task_type": "thanks_task", |
|||
"uuid": uuid, |
|||
"main_parameter": [ |
|||
title, |
|||
config.thank_prompt |
|||
] |
|||
} |
|||
|
|||
references_task = { |
|||
"task_type": "references_task", |
|||
"uuid": uuid, |
|||
"main_parameter": [ |
|||
title, |
|||
mulu, |
|||
config.references_prompt |
|||
] |
|||
} |
|||
|
|||
small_task_list.append(thanks_task) |
|||
small_task_list.append(references_task) |
|||
|
|||
res = { |
|||
"num_small_task": len(small_task_list), |
|||
"tasking_num": 0, |
|||
"标题": title, |
|||
"目录": mulu, |
|||
"开题报告": "", |
|||
"任务书": "", |
|||
"中文摘要": "", |
|||
"英文摘要": "", |
|||
"中文关键词": "", |
|||
"英文关键词": "", |
|||
"正文": "", |
|||
"致谢": "", |
|||
"参考文献": "", |
|||
"table_of_contents": [""] * len(table_of_contents_new) |
|||
} |
|||
|
|||
for small_task in small_task_list: |
|||
result, task_type = small_title_tesk(small_task) |
|||
|
|||
if task_type == "kaitibaogao": |
|||
res["开题报告"] = result |
|||
|
|||
elif task_type == "chat_abstract": |
|||
for i in result: |
|||
res[i] = result[i] |
|||
|
|||
elif task_type == "paper_content": |
|||
content_index = small_task["main_parameter"][0] |
|||
res["table_of_contents"][content_index] = result |
|||
|
|||
elif task_type == "thanks_task": |
|||
res["致谢"] = result |
|||
|
|||
elif task_type == "references_task": |
|||
res["参考文献"] = result |
|||
|
|||
return res |
|||
|
|||
|
|||
def classify(): # 调用模型,设置最大batch_size |
|||
while True: |
|||
if redis_.llen(redis_title) == 0: # 若队列中没有元素就继续获取 |
|||
time.sleep(3) |
|||
continue |
|||
query = redis_.lpop(redis_title).decode('UTF-8') # 获取query的text |
|||
query = json.loads(query) |
|||
|
|||
uuid = query['id'] |
|||
texts = query["text"] |
|||
|
|||
response = main_prrcess(texts) |
|||
print("res", response) |
|||
return_text = str({"texts": response, "probabilities": None, "status_code": 200}) |
|||
|
|||
uuid_path = os.path.join(config.project_data_txt_path, uuid) |
|||
|
|||
os.makedirs(uuid_path) |
|||
|
|||
paper_content_path = os.path.join(uuid_path, "paper_content.json") |
|||
print(uuid) |
|||
with open(paper_content_path, "w") as outfile: |
|||
json.dump(response, outfile) |
|||
|
|||
save_word_paper = os.path.join(uuid_path, "paper.docx") |
|||
save_word_paper_start = os.path.join(uuid_path, "paper_start.docx") |
|||
os.system( |
|||
"java -Dfile.encoding=UTF-8 -jar '/home/majiahui/projert/chatglm/aiXieZuoPro.jar' '{}' '{}' '{}'".format( |
|||
paper_content_path, |
|||
save_word_paper, |
|||
save_word_paper_start)) |
|||
redis_.set(uuid, return_text, 28800) |
|||
|
|||
|
|||
@app.route("/predict", methods=["POST"]) |
|||
def handle_query(): |
|||
print(request.remote_addr) |
|||
texts = request.json["texts"] |
|||
if texts is None: |
|||
return_text = {"texts": "输入了空值", "probabilities": None, "status_code": 402} |
|||
return jsonify(return_text) |
|||
|
|||
id_ = str(uuid.uuid1()) # 为query生成唯一标识 |
|||
d = {'id': id_, 'text': texts} # 绑定文本和query id |
|||
|
|||
redis_.rpush(redis_title, json.dumps(d)) # 加入redis |
|||
while True: |
|||
result = redis_.get(id_) # 获取该query的模型结果 |
|||
if result is not None: |
|||
result_text = {'code': "200", 'data': result.decode('UTF-8')} |
|||
break |
|||
else: |
|||
time.sleep(1) |
|||
|
|||
return jsonify(result_text) # 返回结果 |
|||
|
|||
|
|||
t = Thread(target=classify) |
|||
t.start() |
|||
|
|||
if __name__ == "__main__": |
|||
fh = logging.FileHandler(mode='a', encoding='utf-8', filename='chitchat.log') |
|||
logging.basicConfig( |
|||
handlers=[fh], |
|||
level=logging.DEBUG, |
|||
format='%(asctime)s - %(levelname)s - %(message)s', |
|||
datefmt='%a, %d %b %Y %H:%M:%S', |
|||
) |
|||
app.run(host="0.0.0.0", port=15000, threaded=True, debug=False) |
@ -0,0 +1,43 @@ |
|||
import time |
|||
|
|||
from vllm import LLM, SamplingParams |
|||
|
|||
prompts = [ |
|||
"生成论文小标题内容#问:论文题目是“大学生村官管理研究”,目录是“一、大学生村官管理现状分析\\n1.1 村官数量及分布情况\\n1.2 村官岗位设置及职责\\n1.3 村官工作绩效评估\\n\\n二、大学生村官管理存在的问题\\n2.1 村官队伍结构不合理\\n2.2 村官工作能力不足\\n2.3 村官管理制度不健全\\n\\n三、大学生村官管理对策研究\\n3.1 加强村官队伍建设\\n3.2 提高村官工作能力\\n3.3 完善村官管理制度\\n\\n四、大学生村官管理案例分析\\n4.1 案例一:某村大学生村官工作情况分析\\n4.2 案例二:某村大学生村官管理策略探讨\\n\\n五、大学生村官管理的未来发展趋势\\n5.1 多元化村官队伍建设\\n5.2 信息化村官管理模式\\n5.3 村官职业化发展\\n\\n六、大学生村官管理的政策建议\\n6.1 加强对大学生村官的培训和管理\\n6.2 完善大学生村官管理制度\\n6.3 提高大学生村官的待遇和福利\\n\\n七、结论与展望”,请把其中的小标题“3.3 完善村官管理制度”的内容补充完整,补充内容字数在1500字左右\n答:\n" |
|||
] |
|||
|
|||
# prompts = [ |
|||
# "问:请列出张仲景的所有经方名称\n答:\n" |
|||
# ] |
|||
|
|||
sampling_params = SamplingParams(temperature=0.95, top_p=0.7,presence_penalty=0.9,stop="</s>", max_tokens=2048) |
|||
|
|||
models_path = "/home/majiahui/project/models-llm/openbuddy-llama-7b-finetune" |
|||
llm = LLM(model=models_path, tokenizer_mode="slow") |
|||
|
|||
t1 = time.time() |
|||
outputs = llm.generate(prompts, sampling_params) |
|||
|
|||
# Print the outputs. |
|||
zishu = 0 |
|||
# t2 = time.time() |
|||
for i,output in enumerate(outputs): |
|||
generated_text = output.outputs[0].text |
|||
zishu += len(generated_text) |
|||
print("================================================================================") |
|||
print(i) |
|||
print("=================================================================================") |
|||
print(f"Generated text: {generated_text}") |
|||
|
|||
t2 = time.time() |
|||
time_cost = t2-t1 |
|||
print(time_cost) |
|||
print("speed", zishu/time_cost) |
|||
# |
|||
zishu_one = zishu/time_cost |
|||
print(f"speed: {zishu_one} tokens/s") |
|||
# # from vllm import LLM |
|||
# # |
|||
# # llm = LLM(model="/home/majiahui/models-LLM/openbuddy-llama-7b-v1.4-fp16") # Name or path of your model |
|||
# # output = llm.generate("Hello, my name is") |
|||
# # print(output) |
@ -0,0 +1,98 @@ |
|||
from typing import List, Tuple, Union |
|||
|
|||
from transformers import (AutoTokenizer, PreTrainedTokenizer, |
|||
PreTrainedTokenizerFast) |
|||
|
|||
from vllm.logger import init_logger |
|||
|
|||
logger = init_logger(__name__) |
|||
|
|||
# A fast LLaMA tokenizer with the pre-processed `tokenizer.json` file. |
|||
_FAST_LLAMA_TOKENIZER = "hf-internal-testing/llama-tokenizer" |
|||
|
|||
|
|||
def get_tokenizer( |
|||
tokenizer_name: str, |
|||
*args, |
|||
tokenizer_mode: str = "auto", |
|||
**kwargs, |
|||
) -> Union[PreTrainedTokenizer, PreTrainedTokenizerFast]: |
|||
"""Gets a tokenizer for the given model name via Huggingface.""" |
|||
if tokenizer_mode == "slow": |
|||
if kwargs.get("use_fast", False): |
|||
raise ValueError( |
|||
"Cannot use the fast tokenizer in slow tokenizer mode.") |
|||
kwargs["use_fast"] = False |
|||
|
|||
# if "llama" in tokenizer_name.lower() and kwargs.get("use_fast", True): |
|||
# logger.info( |
|||
# "For some LLaMA-based models, initializing the fast tokenizer may " |
|||
# "take a long time. To eliminate the initialization time, consider " |
|||
# f"using '{_FAST_LLAMA_TOKENIZER}' instead of the original " |
|||
# "tokenizer.") |
|||
try: |
|||
tokenizer = AutoTokenizer.from_pretrained(tokenizer_name, *args, |
|||
**kwargs) |
|||
except TypeError as e: |
|||
# The LLaMA tokenizer causes a protobuf error in some environments. |
|||
err_msg = ( |
|||
"Failed to load the tokenizer. If you are using a LLaMA-based " |
|||
f"model, use '{_FAST_LLAMA_TOKENIZER}' instead of the original " |
|||
"tokenizer.") |
|||
raise RuntimeError(err_msg) from e |
|||
|
|||
if not isinstance(tokenizer, PreTrainedTokenizerFast): |
|||
logger.warning( |
|||
"Using a slow tokenizer. This might cause a significant " |
|||
"slowdown. Consider using a fast tokenizer instead.") |
|||
return tokenizer |
|||
|
|||
|
|||
def detokenize_incrementally( |
|||
tokenizer: Union[PreTrainedTokenizer, PreTrainedTokenizerFast], |
|||
prev_output_tokens: List[str], |
|||
new_token_id: int, |
|||
skip_special_tokens: bool, |
|||
) -> Tuple[str, str]: |
|||
"""Detokenizes the new token in conjuction with the previous output tokens. |
|||
|
|||
NOTE: This function does not update prev_output_tokens. |
|||
|
|||
Returns: |
|||
new_token: The new token as a string. |
|||
output_text: The new output text as a string. |
|||
""" |
|||
new_token = tokenizer.convert_ids_to_tokens( |
|||
new_token_id, skip_special_tokens=skip_special_tokens) |
|||
output_tokens = prev_output_tokens + [new_token] |
|||
|
|||
# Convert the tokens to a string. |
|||
# Optimization: If the tokenizer does not have `added_tokens_encoder`, |
|||
# then we can directly use `convert_tokens_to_string`. |
|||
if not getattr(tokenizer, "added_tokens_encoder", {}): |
|||
output_text = tokenizer.convert_tokens_to_string(output_tokens) |
|||
return new_token, output_text |
|||
|
|||
# Adapted from |
|||
# https://github.com/huggingface/transformers/blob/v4.28.0/src/transformers/tokenization_utils.py#L921 |
|||
# NOTE(woosuk): The following code is slow because it runs a for loop over |
|||
# the output_tokens. In Python, running a for loop over a list can be slow |
|||
# even when the loop body is very simple. |
|||
sub_texts = [] |
|||
current_sub_text = [] |
|||
for token in output_tokens: |
|||
if skip_special_tokens and token in tokenizer.all_special_ids: |
|||
continue |
|||
if token in tokenizer.added_tokens_encoder: |
|||
if current_sub_text: |
|||
sub_text = tokenizer.convert_tokens_to_string(current_sub_text) |
|||
sub_texts.append(sub_text) |
|||
current_sub_text = [] |
|||
sub_texts.append(token) |
|||
else: |
|||
current_sub_text.append(token) |
|||
if current_sub_text: |
|||
sub_text = tokenizer.convert_tokens_to_string(current_sub_text) |
|||
sub_texts.append(sub_text) |
|||
output_text = " ".join(sub_texts) |
|||
return new_token, output_text |
Loading…
Reference in new issue