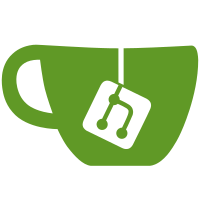
commit
13d84d1f4f
17 changed files with 29191 additions and 0 deletions
File diff suppressed because it is too large
File diff suppressed because it is too large
@ -0,0 +1,722 @@ |
|||
#!/usr/bin/env python |
|||
# coding=utf-8 |
|||
# Copyright 2020 The HuggingFace Inc. team. All rights reserved. |
|||
# |
|||
# Licensed under the Apache License, Version 2.0 (the "License"); |
|||
# you may not use this file except in compliance with the License. |
|||
# You may obtain a copy of the License at |
|||
# |
|||
# http://www.apache.org/licenses/LICENSE-2.0 |
|||
# |
|||
# Unless required by applicable law or agreed to in writing, software |
|||
# distributed under the License is distributed on an "AS IS" BASIS, |
|||
# WITHOUT WARRANTIES OR CONDITIONS OF ANY KIND, either express or implied. |
|||
# See the License for the specific language governing permissions and |
|||
# limitations under the License. |
|||
"""Finetuning the library models for sequence classification on GLUE.""" |
|||
# You can also adapt this script on your own text classification task. Pointers for this are left as comments. |
|||
|
|||
import os |
|||
import re |
|||
|
|||
os.environ["WANDB_DISABLED"] = "true" |
|||
|
|||
# 设置CUDA设备 |
|||
os.environ['CUDA_VISIBLE_DEVICES'] = '2' |
|||
|
|||
|
|||
import logging |
|||
import os |
|||
import random |
|||
import sys |
|||
from dataclasses import dataclass, field |
|||
from typing import Optional |
|||
|
|||
import datasets |
|||
import evaluate |
|||
import numpy as np |
|||
from datasets import load_dataset |
|||
|
|||
import transformers |
|||
from transformers import ( |
|||
AutoConfig, |
|||
AutoModelForSequenceClassification, |
|||
AutoTokenizer, |
|||
DataCollatorWithPadding, |
|||
EvalPrediction, |
|||
HfArgumentParser, |
|||
PretrainedConfig, |
|||
Trainer, |
|||
TrainingArguments, |
|||
default_data_collator, |
|||
set_seed, |
|||
BertTokenizer, |
|||
BertModel |
|||
) |
|||
from transformers import BigBirdModel |
|||
from transformers.trainer_utils import get_last_checkpoint |
|||
from transformers.utils import check_min_version, send_example_telemetry |
|||
from transformers.utils.versions import require_version |
|||
|
|||
from BertClsModel import BertForSequenceClassification |
|||
|
|||
# Will error if the minimal version of Transformers is not installed. Remove at your own risks. |
|||
# check_min_version("4.43.0.dev0") |
|||
|
|||
require_version("datasets>=1.8.0", "To fix: pip install -r examples/pytorch/text-classification/requirements.txt") |
|||
|
|||
task_to_keys = { |
|||
"cola": ("sentence", None), |
|||
"mnli": ("premise", "hypothesis"), |
|||
"mrpc": ("sentence1", "sentence2"), |
|||
"qnli": ("question", "sentence"), |
|||
"qqp": ("question1", "question2"), |
|||
"rte": ("sentence1", "sentence2"), |
|||
"sst2": ("sentence", None), |
|||
"stsb": ("sentence1", "sentence2"), |
|||
"wnli": ("sentence1", "sentence2"), |
|||
} |
|||
|
|||
logger = logging.getLogger(__name__) |
|||
|
|||
|
|||
@dataclass |
|||
class DataTrainingArguments: |
|||
""" |
|||
Arguments pertaining to what data we are going to input our model for training and eval. |
|||
|
|||
Using `HfArgumentParser` we can turn this class |
|||
into argparse arguments to be able to specify them on |
|||
the command line. |
|||
""" |
|||
|
|||
task_name: Optional[str] = field( |
|||
default=None, |
|||
metadata={"help": "The name of the task to train on: " + ", ".join(task_to_keys.keys())}, |
|||
) |
|||
dataset_name: Optional[str] = field( |
|||
default=None, metadata={"help": "The name of the dataset to use (via the datasets library)."} |
|||
) |
|||
dataset_config_name: Optional[str] = field( |
|||
default=None, metadata={"help": "The configuration name of the dataset to use (via the datasets library)."} |
|||
) |
|||
max_seq_length: int = field( |
|||
default=128, |
|||
metadata={ |
|||
"help": ( |
|||
"The maximum total input sequence length after tokenization. Sequences longer " |
|||
"than this will be truncated, sequences shorter will be padded." |
|||
) |
|||
}, |
|||
) |
|||
overwrite_cache: bool = field( |
|||
default=False, metadata={"help": "Overwrite the cached preprocessed datasets or not."} |
|||
) |
|||
pad_to_max_length: bool = field( |
|||
default=True, |
|||
metadata={ |
|||
"help": ( |
|||
"Whether to pad all samples to `max_seq_length`. " |
|||
"If False, will pad the samples dynamically when batching to the maximum length in the batch." |
|||
) |
|||
}, |
|||
) |
|||
max_train_samples: Optional[int] = field( |
|||
default=None, |
|||
metadata={ |
|||
"help": ( |
|||
"For debugging purposes or quicker training, truncate the number of training examples to this " |
|||
"value if set." |
|||
) |
|||
}, |
|||
) |
|||
max_eval_samples: Optional[int] = field( |
|||
default=None, |
|||
metadata={ |
|||
"help": ( |
|||
"For debugging purposes or quicker training, truncate the number of evaluation examples to this " |
|||
"value if set." |
|||
) |
|||
}, |
|||
) |
|||
max_predict_samples: Optional[int] = field( |
|||
default=None, |
|||
metadata={ |
|||
"help": ( |
|||
"For debugging purposes or quicker training, truncate the number of prediction examples to this " |
|||
"value if set." |
|||
) |
|||
}, |
|||
) |
|||
train_file: Optional[str] = field( |
|||
default=None, metadata={"help": "A csv or a json file containing the training data."} |
|||
) |
|||
validation_file: Optional[str] = field( |
|||
default=None, metadata={"help": "A csv or a json file containing the validation data."} |
|||
) |
|||
test_file: Optional[str] = field(default=None, metadata={"help": "A csv or a json file containing the test data."}) |
|||
|
|||
def __post_init__(self): |
|||
if self.task_name is not None: |
|||
self.task_name = self.task_name.lower() |
|||
if self.task_name not in task_to_keys.keys(): |
|||
raise ValueError("Unknown task, you should pick one in " + ",".join(task_to_keys.keys())) |
|||
elif self.dataset_name is not None: |
|||
pass |
|||
elif self.train_file is None or self.validation_file is None: |
|||
raise ValueError("Need either a GLUE task, a training/validation file or a dataset name.") |
|||
else: |
|||
train_extension = self.train_file.split(".")[-1] |
|||
assert train_extension in ["csv", "json"], "`train_file` should be a csv or a json file." |
|||
validation_extension = self.validation_file.split(".")[-1] |
|||
assert ( |
|||
validation_extension == train_extension |
|||
), "`validation_file` should have the same extension (csv or json) as `train_file`." |
|||
|
|||
|
|||
@dataclass |
|||
class ModelArguments: |
|||
""" |
|||
Arguments pertaining to which model/config/tokenizer we are going to fine-tune from. |
|||
""" |
|||
|
|||
model_name_or_path: str = field( |
|||
metadata={"help": "Path to pretrained model or model identifier from huggingface.co/models"} |
|||
) |
|||
config_name: Optional[str] = field( |
|||
default=None, metadata={"help": "Pretrained config name or path if not the same as model_name"} |
|||
) |
|||
tokenizer_name: Optional[str] = field( |
|||
default=None, metadata={"help": "Pretrained tokenizer name or path if not the same as model_name"} |
|||
) |
|||
cache_dir: Optional[str] = field( |
|||
default=None, |
|||
metadata={"help": "Where do you want to store the pretrained models downloaded from huggingface.co"}, |
|||
) |
|||
use_fast_tokenizer: bool = field( |
|||
default=True, |
|||
metadata={"help": "Whether to use one of the fast tokenizer (backed by the tokenizers library) or not."}, |
|||
) |
|||
model_revision: str = field( |
|||
default="main", |
|||
metadata={"help": "The specific model version to use (can be a branch name, tag name or commit id)."}, |
|||
) |
|||
token: str = field( |
|||
default=None, |
|||
metadata={ |
|||
"help": ( |
|||
"The token to use as HTTP bearer authorization for remote files. If not specified, will use the token " |
|||
"generated when running `huggingface-cli login` (stored in `~/.huggingface`)." |
|||
) |
|||
}, |
|||
) |
|||
trust_remote_code: bool = field( |
|||
default=False, |
|||
metadata={ |
|||
"help": ( |
|||
"Whether to trust the execution of code from datasets/models defined on the Hub." |
|||
" This option should only be set to `True` for repositories you trust and in which you have read the" |
|||
" code, as it will execute code present on the Hub on your local machine." |
|||
) |
|||
}, |
|||
) |
|||
ignore_mismatched_sizes: bool = field( |
|||
default=False, |
|||
metadata={"help": "Will enable to load a pretrained model whose head dimensions are different."}, |
|||
) |
|||
|
|||
def data_padding(data_list, max_seq_length): |
|||
data_list_new = [] |
|||
for dan_sentence in data_list: |
|||
padding_list = [0] * (max_seq_length - len(dan_sentence)) |
|||
dan_sentence.extend(padding_list) |
|||
data_list_new.append(dan_sentence) |
|||
print("dan_sentence", len(dan_sentence)) |
|||
print("=================================") |
|||
return data_list_new |
|||
|
|||
|
|||
def tokenizer_ulit(tokenizer, data_list, padding, max_seq_length): |
|||
pattern = r"<\\Start>.*<\\End>" |
|||
# 7549 |
|||
zhanweifu = "顯顯顯顯" |
|||
content_t_list = [] |
|||
biaoji_list = [] |
|||
for i in range(len(data_list)): |
|||
biaoji = re.findall(pattern, data_list[i], re.DOTALL)[0] |
|||
content_t = data_list[i].replace(biaoji, zhanweifu) |
|||
content_t_list.append(content_t) |
|||
biaoji_list.append(biaoji) |
|||
|
|||
result_content_t_list = tokenizer(content_t_list, truncation=True) |
|||
result_biaoji_list = tokenizer(biaoji_list, truncation=True) |
|||
|
|||
# print("result_content_t", result_content_t_list) |
|||
# print("result_biaoji", result_biaoji_list) |
|||
|
|||
for index_content in range(len(result_content_t_list['input_ids'])): |
|||
result_biaoji_list['input_ids'][index_content] = result_biaoji_list['input_ids'][index_content][1:-1] |
|||
len_biaoji = len(result_biaoji_list['input_ids'][index_content]) |
|||
result_biaoji_list['token_type_ids'][index_content] = [1] * len_biaoji |
|||
result_biaoji_list['attention_mask'][index_content] = [1] * len_biaoji |
|||
|
|||
index_ = result_content_t_list['input_ids'][index_content].index(7549) |
|||
|
|||
for _ in range(index_, index_ + 4): # 循环删除下标3, 4, 5的元素 |
|||
result_content_t_list['input_ids'][index_content].pop(index_) |
|||
|
|||
for _ in range(index_, index_ + 4): # 循环删除下标3, 4, 5的元素 |
|||
result_content_t_list['token_type_ids'][index_content].pop(index_) |
|||
|
|||
for _ in range(index_, index_ + 4): # 循环删除下标3, 4, 5的元素 |
|||
result_content_t_list['attention_mask'][index_content].pop(index_) |
|||
|
|||
for i in range(len(result_biaoji_list['input_ids'][index_content])): |
|||
result_content_t_list['input_ids'][index_content].insert(index_ + i, |
|||
result_biaoji_list['input_ids'][index_content][i]) |
|||
|
|||
for i in range(len(result_biaoji_list['token_type_ids'][index_content])): |
|||
result_content_t_list['token_type_ids'][index_content].insert(index_ + i, |
|||
result_biaoji_list['token_type_ids'][ |
|||
index_content][i]) |
|||
|
|||
for i in range(len(result_biaoji_list['attention_mask'][index_content])): |
|||
result_content_t_list['attention_mask'][index_content].insert(index_ + i, |
|||
result_biaoji_list['attention_mask'][ |
|||
index_content][i]) |
|||
|
|||
result_content_t_list['input_ids'] = data_padding(result_content_t_list['input_ids'], max_seq_length=2048) |
|||
result_content_t_list['token_type_ids'] = data_padding(result_content_t_list['token_type_ids'], max_seq_length=2048) |
|||
result_content_t_list['attention_mask'] = data_padding(result_content_t_list['attention_mask'], max_seq_length=2048) |
|||
return result_content_t_list |
|||
|
|||
def main(): |
|||
# See all possible arguments in src/transformers/training_args.py |
|||
# or by passing the --help flag to this script. |
|||
# We now keep distinct sets of args, for a cleaner separation of concerns. |
|||
|
|||
parser = HfArgumentParser((ModelArguments, DataTrainingArguments, TrainingArguments)) |
|||
if len(sys.argv) == 2 and sys.argv[1].endswith(".json"): |
|||
# If we pass only one argument to the script and it's the path to a json file, |
|||
# let's parse it to get our arguments. |
|||
model_args, data_args, training_args = parser.parse_json_file(json_file=os.path.abspath(sys.argv[1])) |
|||
else: |
|||
model_args, data_args, training_args = parser.parse_args_into_dataclasses() |
|||
|
|||
# Sending telemetry. Tracking the example usage helps us better allocate resources to maintain them. The |
|||
# information sent is the one passed as arguments along with your Python/PyTorch versions. |
|||
send_example_telemetry("run_glue", model_args, data_args) |
|||
print("model_args", model_args) |
|||
9/0 |
|||
|
|||
# Setup logging |
|||
logging.basicConfig( |
|||
format="%(asctime)s - %(levelname)s - %(name)s - %(message)s", |
|||
datefmt="%m/%d/%Y %H:%M:%S", |
|||
handlers=[logging.StreamHandler(sys.stdout)], |
|||
) |
|||
|
|||
if training_args.should_log: |
|||
# The default of training_args.log_level is passive, so we set log level at info here to have that default. |
|||
transformers.utils.logging.set_verbosity_info() |
|||
|
|||
log_level = training_args.get_process_log_level() |
|||
logger.setLevel(log_level) |
|||
datasets.utils.logging.set_verbosity(log_level) |
|||
transformers.utils.logging.set_verbosity(log_level) |
|||
transformers.utils.logging.enable_default_handler() |
|||
transformers.utils.logging.enable_explicit_format() |
|||
|
|||
# Log on each process the small summary: |
|||
logger.warning( |
|||
f"Process rank: {training_args.local_rank}, device: {training_args.device}, n_gpu: {training_args.n_gpu}, " |
|||
+ f"distributed training: {training_args.parallel_mode.value == 'distributed'}, 16-bits training: {training_args.fp16}" |
|||
) |
|||
logger.info(f"Training/evaluation parameters {training_args}") |
|||
|
|||
# Detecting last checkpoint. |
|||
last_checkpoint = None |
|||
if os.path.isdir(training_args.output_dir) and training_args.do_train and not training_args.overwrite_output_dir: |
|||
last_checkpoint = get_last_checkpoint(training_args.output_dir) |
|||
if last_checkpoint is None and len(os.listdir(training_args.output_dir)) > 0: |
|||
raise ValueError( |
|||
f"Output directory ({training_args.output_dir}) already exists and is not empty. " |
|||
"Use --overwrite_output_dir to overcome." |
|||
) |
|||
elif last_checkpoint is not None and training_args.resume_from_checkpoint is None: |
|||
logger.info( |
|||
f"Checkpoint detected, resuming training at {last_checkpoint}. To avoid this behavior, change " |
|||
"the `--output_dir` or add `--overwrite_output_dir` to train from scratch." |
|||
) |
|||
|
|||
# Set seed before initializing model. |
|||
set_seed(training_args.seed) |
|||
|
|||
# Get the datasets: you can either provide your own CSV/JSON training and evaluation files (see below) |
|||
# or specify a GLUE benchmark task (the dataset will be downloaded automatically from the datasets Hub). |
|||
# |
|||
# For CSV/JSON files, this script will use as labels the column called 'label' and as pair of sentences the |
|||
# sentences in columns called 'sentence1' and 'sentence2' if such column exists or the first two columns not named |
|||
# label if at least two columns are provided. |
|||
# |
|||
# If the CSVs/JSONs contain only one non-label column, the script does single sentence classification on this |
|||
# single column. You can easily tweak this behavior (see below) |
|||
# |
|||
# In distributed training, the load_dataset function guarantee that only one local process can concurrently |
|||
# download the dataset. |
|||
if data_args.task_name is not None: |
|||
# Downloading and loading a dataset from the hub. |
|||
raw_datasets = load_dataset( |
|||
"nyu-mll/glue", |
|||
data_args.task_name, |
|||
cache_dir=model_args.cache_dir, |
|||
token=model_args.token, |
|||
) |
|||
elif data_args.dataset_name is not None: |
|||
# Downloading and loading a dataset from the hub. |
|||
raw_datasets = load_dataset( |
|||
data_args.dataset_name, |
|||
data_args.dataset_config_name, |
|||
cache_dir=model_args.cache_dir, |
|||
token=model_args.token, |
|||
trust_remote_code=model_args.trust_remote_code, |
|||
) |
|||
else: |
|||
# Loading a dataset from your local files. |
|||
# CSV/JSON training and evaluation files are needed. |
|||
data_files = {"train": data_args.train_file, "validation": data_args.validation_file} |
|||
|
|||
# Get the test dataset: you can provide your own CSV/JSON test file (see below) |
|||
# when you use `do_predict` without specifying a GLUE benchmark task. |
|||
if training_args.do_predict: |
|||
if data_args.test_file is not None: |
|||
train_extension = data_args.train_file.split(".")[-1] |
|||
test_extension = data_args.test_file.split(".")[-1] |
|||
assert ( |
|||
test_extension == train_extension |
|||
), "`test_file` should have the same extension (csv or json) as `train_file`." |
|||
data_files["test"] = data_args.test_file |
|||
else: |
|||
raise ValueError("Need either a GLUE task or a test file for `do_predict`.") |
|||
|
|||
for key in data_files.keys(): |
|||
logger.info(f"load a local file for {key}: {data_files[key]}") |
|||
|
|||
if data_args.train_file.endswith(".csv"): |
|||
# Loading a dataset from local csv files |
|||
raw_datasets = load_dataset( |
|||
"csv", |
|||
data_files=data_files, |
|||
cache_dir=model_args.cache_dir, |
|||
token=model_args.token, |
|||
) |
|||
else: |
|||
# Loading a dataset from local json files |
|||
raw_datasets = load_dataset( |
|||
"json", |
|||
data_files=data_files, |
|||
cache_dir=model_args.cache_dir, |
|||
token=model_args.token, |
|||
) |
|||
# See more about loading any type of standard or custom dataset at |
|||
# https://huggingface.co/docs/datasets/loading_datasets. |
|||
|
|||
# Labels |
|||
if data_args.task_name is not None: |
|||
is_regression = data_args.task_name == "stsb" |
|||
if not is_regression: |
|||
label_list = raw_datasets["train"].features["label"].names |
|||
num_labels = len(label_list) |
|||
else: |
|||
num_labels = 1 |
|||
else: |
|||
# Trying to have good defaults here, don't hesitate to tweak to your needs. |
|||
is_regression = raw_datasets["train"].features["label"].dtype in ["float32", "float64"] |
|||
if is_regression: |
|||
num_labels = 1 |
|||
else: |
|||
# A useful fast method: |
|||
# https://huggingface.co/docs/datasets/package_reference/main_classes#datasets.Dataset.unique |
|||
label_list = raw_datasets["train"].unique("label") |
|||
label_list.sort() # Let's sort it for determinism |
|||
num_labels = len(label_list) |
|||
|
|||
# Load pretrained model and tokenizer |
|||
# |
|||
# In distributed training, the .from_pretrained methods guarantee that only one local process can concurrently |
|||
# download model & vocab. |
|||
print(num_labels) |
|||
config = AutoConfig.from_pretrained( |
|||
model_args.config_name if model_args.config_name else model_args.model_name_or_path, |
|||
num_labels=num_labels, |
|||
finetuning_task=data_args.task_name, |
|||
cache_dir=model_args.cache_dir, |
|||
revision=model_args.model_revision, |
|||
token=model_args.token, |
|||
trust_remote_code=model_args.trust_remote_code, |
|||
) |
|||
tokenizer = BertTokenizer.from_pretrained( |
|||
model_args.tokenizer_name if model_args.tokenizer_name else model_args.model_name_or_path, |
|||
cache_dir=model_args.cache_dir, |
|||
use_fast=model_args.use_fast_tokenizer, |
|||
revision=model_args.model_revision, |
|||
token=model_args.token, |
|||
trust_remote_code=model_args.trust_remote_code, |
|||
) |
|||
model = BertForSequenceClassification.from_pretrained( |
|||
model_args.model_name_or_path, |
|||
from_tf=bool(".ckpt" in model_args.model_name_or_path), |
|||
config=config, |
|||
cache_dir=model_args.cache_dir, |
|||
revision=model_args.model_revision, |
|||
token=model_args.token, |
|||
trust_remote_code=model_args.trust_remote_code, |
|||
ignore_mismatched_sizes=model_args.ignore_mismatched_sizes, |
|||
) |
|||
|
|||
# Preprocessing the raw_datasets |
|||
if data_args.task_name is not None: |
|||
sentence1_key, sentence2_key = task_to_keys[data_args.task_name] |
|||
else: |
|||
# Again, we try to have some nice defaults but don't hesitate to tweak to your use case. |
|||
non_label_column_names = [name for name in raw_datasets["train"].column_names if name != "label"] |
|||
if "sentence1" in non_label_column_names and "sentence2" in non_label_column_names: |
|||
sentence1_key, sentence2_key = "sentence1", "sentence2" |
|||
else: |
|||
if len(non_label_column_names) >= 2: |
|||
sentence1_key, sentence2_key = non_label_column_names[:2] |
|||
else: |
|||
sentence1_key, sentence2_key = non_label_column_names[0], None |
|||
|
|||
# Padding strategy |
|||
if data_args.pad_to_max_length: |
|||
padding = "max_length" |
|||
else: |
|||
# We will pad later, dynamically at batch creation, to the max sequence length in each batch |
|||
padding = False |
|||
|
|||
# Some models have set the order of the labels to use, so let's make sure we do use it. |
|||
label_to_id = None |
|||
if ( |
|||
model.config.label2id != PretrainedConfig(num_labels=num_labels).label2id |
|||
and data_args.task_name is not None |
|||
and not is_regression |
|||
): |
|||
# Some have all caps in their config, some don't. |
|||
label_name_to_id = {k.lower(): v for k, v in model.config.label2id.items()} |
|||
if sorted(label_name_to_id.keys()) == sorted(label_list): |
|||
label_to_id = {i: int(label_name_to_id[label_list[i]]) for i in range(num_labels)} |
|||
else: |
|||
logger.warning( |
|||
"Your model seems to have been trained with labels, but they don't match the dataset: ", |
|||
f"model labels: {sorted(label_name_to_id.keys())}, dataset labels: {sorted(label_list)}." |
|||
"\nIgnoring the model labels as a result.", |
|||
) |
|||
elif data_args.task_name is None and not is_regression: |
|||
label_to_id = {v: i for i, v in enumerate(label_list)} |
|||
|
|||
if label_to_id is not None: |
|||
model.config.label2id = label_to_id |
|||
model.config.id2label = {id: label for label, id in config.label2id.items()} |
|||
elif data_args.task_name is not None and not is_regression: |
|||
model.config.label2id = {l: i for i, l in enumerate(label_list)} |
|||
model.config.id2label = {id: label for label, id in config.label2id.items()} |
|||
|
|||
if data_args.max_seq_length > tokenizer.model_max_length: |
|||
logger.warning( |
|||
f"The max_seq_length passed ({data_args.max_seq_length}) is larger than the maximum length for the " |
|||
f"model ({tokenizer.model_max_length}). Using max_seq_length={tokenizer.model_max_length}." |
|||
) |
|||
max_seq_length = min(data_args.max_seq_length, tokenizer.model_max_length) |
|||
|
|||
def preprocess_function(examples): |
|||
print(examples) |
|||
print("1") |
|||
# print("examples[sentence1_key]", examples[sentence1_key]) |
|||
# print("len(examples[sentence1_key])", len(examples[sentence1_key])) |
|||
# print("padding", padding) |
|||
# Tokenize the texts |
|||
args = ( |
|||
(examples[sentence1_key],) if sentence2_key is None else (examples[sentence1_key], examples[sentence2_key]) |
|||
) |
|||
result = tokenizer(*args, padding=padding, max_length=max_seq_length, truncation=True) |
|||
# result = tokenizer_ulit(tokenizer, examples[sentence1_key], padding, max_seq_length) |
|||
# Map labels to IDs (not necessary for GLUE tasks) |
|||
if label_to_id is not None and "label" in examples: |
|||
result["label"] = [(label_to_id[l] if l != -1 else -1) for l in examples["label"]] |
|||
return result |
|||
|
|||
with training_args.main_process_first(desc="dataset map pre-processing"): |
|||
raw_datasets = raw_datasets.map( |
|||
preprocess_function, |
|||
batched=True, |
|||
load_from_cache_file=not data_args.overwrite_cache, |
|||
desc="Running tokenizer on dataset", |
|||
) |
|||
if training_args.do_train: |
|||
if "train" not in raw_datasets: |
|||
raise ValueError("--do_train requires a train dataset") |
|||
train_dataset = raw_datasets["train"] |
|||
if data_args.max_train_samples is not None: |
|||
max_train_samples = min(len(train_dataset), data_args.max_train_samples) |
|||
train_dataset = train_dataset.select(range(max_train_samples)) |
|||
|
|||
if training_args.do_eval: |
|||
if "validation" not in raw_datasets and "validation_matched" not in raw_datasets: |
|||
raise ValueError("--do_eval requires a validation dataset") |
|||
eval_dataset = raw_datasets["validation_matched" if data_args.task_name == "mnli" else "validation"] |
|||
if data_args.max_eval_samples is not None: |
|||
max_eval_samples = min(len(eval_dataset), data_args.max_eval_samples) |
|||
eval_dataset = eval_dataset.select(range(max_eval_samples)) |
|||
|
|||
if training_args.do_predict or data_args.task_name is not None or data_args.test_file is not None: |
|||
if "test" not in raw_datasets and "test_matched" not in raw_datasets: |
|||
raise ValueError("--do_predict requires a test dataset") |
|||
predict_dataset = raw_datasets["test_matched" if data_args.task_name == "mnli" else "test"] |
|||
if data_args.max_predict_samples is not None: |
|||
max_predict_samples = min(len(predict_dataset), data_args.max_predict_samples) |
|||
predict_dataset = predict_dataset.select(range(max_predict_samples)) |
|||
|
|||
# Log a few random samples from the training set: |
|||
if training_args.do_train: |
|||
for index in random.sample(range(len(train_dataset)), 3): |
|||
logger.info(f"Sample {index} of the training set: {train_dataset[index]}.") |
|||
|
|||
# Get the metric function |
|||
if data_args.task_name is not None: |
|||
metric = evaluate.load("glue", data_args.task_name, cache_dir=model_args.cache_dir) |
|||
elif is_regression: |
|||
metric = evaluate.load("mse", cache_dir=model_args.cache_dir) |
|||
else: |
|||
metric = evaluate.load("./metrics/accuracy", cache_dir=model_args.cache_dir) |
|||
|
|||
# You can define your custom compute_metrics function. It takes an `EvalPrediction` object (a namedtuple with a |
|||
# predictions and label_ids field) and has to return a dictionary string to float. |
|||
def compute_metrics(p: EvalPrediction): |
|||
preds = p.predictions[0] if isinstance(p.predictions, tuple) else p.predictions |
|||
preds = np.squeeze(preds) if is_regression else np.argmax(preds, axis=1) |
|||
result = metric.compute(predictions=preds, references=p.label_ids) |
|||
if len(result) > 1: |
|||
result["combined_score"] = np.mean(list(result.values())).item() |
|||
return result |
|||
|
|||
# Data collator will default to DataCollatorWithPadding when the tokenizer is passed to Trainer, so we change it if |
|||
# we already did the padding. |
|||
if data_args.pad_to_max_length: |
|||
data_collator = default_data_collator |
|||
elif training_args.fp16: |
|||
data_collator = DataCollatorWithPadding(tokenizer, pad_to_multiple_of=8) |
|||
else: |
|||
data_collator = None |
|||
|
|||
# Initialize our Trainer |
|||
trainer = Trainer( |
|||
model=model, |
|||
args=training_args, |
|||
train_dataset=train_dataset if training_args.do_train else None, |
|||
eval_dataset=eval_dataset if training_args.do_eval else None, |
|||
compute_metrics=compute_metrics, |
|||
tokenizer=tokenizer, |
|||
data_collator=data_collator, |
|||
) |
|||
|
|||
# Training |
|||
if training_args.do_train: |
|||
checkpoint = None |
|||
if training_args.resume_from_checkpoint is not None: |
|||
checkpoint = training_args.resume_from_checkpoint |
|||
elif last_checkpoint is not None: |
|||
checkpoint = last_checkpoint |
|||
train_result = trainer.train(resume_from_checkpoint=checkpoint) |
|||
metrics = train_result.metrics |
|||
max_train_samples = ( |
|||
data_args.max_train_samples if data_args.max_train_samples is not None else len(train_dataset) |
|||
) |
|||
metrics["train_samples"] = min(max_train_samples, len(train_dataset)) |
|||
|
|||
trainer.save_model() # Saves the tokenizer too for easy upload |
|||
|
|||
trainer.log_metrics("train", metrics) |
|||
trainer.save_metrics("train", metrics) |
|||
trainer.save_state() |
|||
|
|||
# Evaluation |
|||
if training_args.do_eval: |
|||
logger.info("*** Evaluate ***") |
|||
|
|||
# Loop to handle MNLI double evaluation (matched, mis-matched) |
|||
tasks = [data_args.task_name] |
|||
eval_datasets = [eval_dataset] |
|||
if data_args.task_name == "mnli": |
|||
tasks.append("mnli-mm") |
|||
valid_mm_dataset = raw_datasets["validation_mismatched"] |
|||
if data_args.max_eval_samples is not None: |
|||
max_eval_samples = min(len(valid_mm_dataset), data_args.max_eval_samples) |
|||
valid_mm_dataset = valid_mm_dataset.select(range(max_eval_samples)) |
|||
eval_datasets.append(valid_mm_dataset) |
|||
combined = {} |
|||
|
|||
for eval_dataset, task in zip(eval_datasets, tasks): |
|||
metrics = trainer.evaluate(eval_dataset=eval_dataset) |
|||
|
|||
max_eval_samples = ( |
|||
data_args.max_eval_samples if data_args.max_eval_samples is not None else len(eval_dataset) |
|||
) |
|||
metrics["eval_samples"] = min(max_eval_samples, len(eval_dataset)) |
|||
|
|||
if task == "mnli-mm": |
|||
metrics = {k + "_mm": v for k, v in metrics.items()} |
|||
if task is not None and "mnli" in task: |
|||
combined.update(metrics) |
|||
|
|||
trainer.log_metrics("eval", metrics) |
|||
trainer.save_metrics("eval", combined if task is not None and "mnli" in task else metrics) |
|||
|
|||
if training_args.do_predict: |
|||
logger.info("*** Predict ***") |
|||
|
|||
# Loop to handle MNLI double evaluation (matched, mis-matched) |
|||
tasks = [data_args.task_name] |
|||
predict_datasets = [predict_dataset] |
|||
if data_args.task_name == "mnli": |
|||
tasks.append("mnli-mm") |
|||
predict_datasets.append(raw_datasets["test_mismatched"]) |
|||
|
|||
for predict_dataset, task in zip(predict_datasets, tasks): |
|||
# Removing the `label` columns because it contains -1 and Trainer won't like that. |
|||
predict_dataset = predict_dataset.remove_columns("label") |
|||
predictions = trainer.predict(predict_dataset, metric_key_prefix="predict").predictions |
|||
predictions = np.squeeze(predictions) if is_regression else np.argmax(predictions, axis=1) |
|||
|
|||
output_predict_file = os.path.join(training_args.output_dir, f"predict_results_{task}.txt") |
|||
if trainer.is_world_process_zero(): |
|||
with open(output_predict_file, "w") as writer: |
|||
logger.info(f"***** Predict results {task} *****") |
|||
writer.write("index\tprediction\n") |
|||
for index, item in enumerate(predictions): |
|||
if is_regression: |
|||
writer.write(f"{index}\t{item:3.3f}\n") |
|||
else: |
|||
item = label_list[item] |
|||
writer.write(f"{index}\t{item}\n") |
|||
|
|||
kwargs = {"finetuned_from": model_args.model_name_or_path, "tasks": "text-classification"} |
|||
if data_args.task_name is not None: |
|||
kwargs["language"] = "en" |
|||
kwargs["dataset_tags"] = "glue" |
|||
kwargs["dataset_args"] = data_args.task_name |
|||
kwargs["dataset"] = f"GLUE {data_args.task_name.upper()}" |
|||
|
|||
if training_args.push_to_hub: |
|||
trainer.push_to_hub(**kwargs) |
|||
else: |
|||
trainer.create_model_card(**kwargs) |
|||
|
|||
|
|||
def _mp_fn(index): |
|||
# For xla_spawn (TPUs) |
|||
main() |
|||
|
|||
|
|||
if __name__ == "__main__": |
|||
main() |
@ -0,0 +1,11 @@ |
|||
python run_glue.py \ |
|||
--model_name_or_path chinese_bert_wwm_ext_pytorch \ |
|||
--train_file data/train_data_weipu.csv \ |
|||
--validation_file data/dev_data_weipu.csv \ |
|||
--do_train \ |
|||
--do_eval \ |
|||
--max_seq_length 512 \ |
|||
--per_device_train_batch_size 32 \ |
|||
--learning_rate 2e-5 \ |
|||
--num_train_epochs 5 \ |
|||
--output_dir aigc_check |
@ -0,0 +1,6 @@ |
|||
from datasets import load_dataset |
|||
|
|||
dataset = load_dataset('json', data_files='data/ceshi_3.json') |
|||
print(dataset) # 检查第一条数据 |
|||
print(dataset["train"][1]) |
|||
print(dataset["train"]) |
@ -0,0 +1,8 @@ |
|||
import pandas as pd |
|||
|
|||
data_1 = pd.read_csv("data/train_1_1_data_weipu.csv.csv").values.tolist() |
|||
data_2 = pd.read_csv("data/train_2_2_data_weipu.csv.csv").values.tolist() |
|||
|
|||
data = data_1 + data_2 |
|||
|
|||
pd.DataFrame(data, columns=["sentence", "label"]).to_csv("data/train_data_weipu_3.csv", index=False) |
@ -0,0 +1,155 @@ |
|||
import json |
|||
import os |
|||
import re |
|||
|
|||
os.environ["WANDB_DISABLED"] = "true" |
|||
|
|||
|
|||
# 设置CUDA设备 |
|||
os.environ['CUDA_VISIBLE_DEVICES'] = '1' |
|||
|
|||
|
|||
import logging |
|||
import os |
|||
import random |
|||
import sys |
|||
from dataclasses import dataclass, field |
|||
from typing import Optional |
|||
|
|||
import datasets |
|||
import evaluate |
|||
import numpy as np |
|||
from datasets import load_dataset |
|||
import torch |
|||
import transformers |
|||
from transformers import ( |
|||
AutoConfig, |
|||
AutoModelForSequenceClassification, |
|||
AutoTokenizer, |
|||
DataCollatorWithPadding, |
|||
EvalPrediction, |
|||
HfArgumentParser, |
|||
PretrainedConfig, |
|||
Trainer, |
|||
TrainingArguments, |
|||
default_data_collator, |
|||
set_seed, |
|||
BertTokenizer, |
|||
BertModel |
|||
) |
|||
from transformers import BigBirdModel |
|||
from transformers.trainer_utils import get_last_checkpoint |
|||
from transformers.utils import check_min_version, send_example_telemetry |
|||
from transformers.utils.versions import require_version |
|||
|
|||
from BertClsModel import BertForSequenceClassification |
|||
import pandas as pd |
|||
from tqdm import tqdm |
|||
|
|||
|
|||
def load_model(model_path: str): |
|||
config = AutoConfig.from_pretrained( |
|||
model_path, |
|||
num_labels=4, |
|||
) |
|||
|
|||
tokenizer = BertTokenizer.from_pretrained( |
|||
model_path |
|||
) |
|||
|
|||
model = BertForSequenceClassification.from_pretrained( |
|||
model_path, |
|||
config=config |
|||
) |
|||
return model, tokenizer |
|||
|
|||
id_2_lable = { |
|||
0: "正文", |
|||
1: "一级标题", |
|||
2: "二级标题", |
|||
3: "三级标题", |
|||
} |
|||
|
|||
|
|||
if __name__ == "__main__": |
|||
model, tokenizer = load_model(model_path='/home/majiahui/project/text-classification-long/long_paper_1') |
|||
|
|||
# text = "(1)经病理学或细胞学确诊的肺癌患者;" |
|||
# |
|||
# sen = [text] |
|||
# result = tokenizer(sen, max_length=512, truncation=True) |
|||
# print(result) |
|||
# |
|||
# input_ids = result['input_ids'] |
|||
# token_type_ids = result['token_type_ids'] |
|||
# |
|||
# input_ids = seq_padding(tokenizer, input_ids) |
|||
# token_type_ids = seq_padding(tokenizer, token_type_ids) |
|||
# |
|||
# |
|||
# result = model(input_ids=input_ids,token_type_ids=token_type_ids) # 这里不需要labels |
|||
# output = torch.sigmoid(result[0][0]).tolist() |
|||
# # result_ = result[0][0] |
|||
# print(output) |
|||
|
|||
model.to("cuda") |
|||
data_list = pd.read_csv("data/long_paper_2.csv").values.tolist() |
|||
data_new = [] |
|||
|
|||
zong = 0 |
|||
rel = 0 |
|||
jishu = 0 |
|||
|
|||
for i in tqdm(data_list): |
|||
# print(zong) |
|||
# print(i) |
|||
zong += 1 |
|||
text = i[0] |
|||
lable = i[1] |
|||
|
|||
result = tokenizer([text], max_length=2048, truncation=True) |
|||
|
|||
input_ids = result['input_ids'] |
|||
token_type_ids = result['token_type_ids'] |
|||
# print(input_ids) |
|||
# print(text) |
|||
# print(lable) |
|||
input_ids = torch.tensor(input_ids) # 将列表转换为 PyTorch tensor |
|||
token_type_ids = torch.tensor(token_type_ids) # 将列表转换为 PyTorch tensor |
|||
input_ids = input_ids.long() |
|||
token_type_ids = token_type_ids.long() |
|||
|
|||
batch_masks = input_ids.gt(0).to("cuda") |
|||
input_ids, token_type_ids = input_ids.to("cuda"), token_type_ids.to("cuda") |
|||
result = model(input_ids=input_ids,token_type_ids=token_type_ids, attention_mask=batch_masks) # 这里不需要labels |
|||
# output = torch.sigmoid(result[0][0]).tolist() |
|||
# # result_ = result[0][0] |
|||
# if output[1] > 0.5: |
|||
# rel += 1 |
|||
# |
|||
# data_new.append({ |
|||
# "index": index, |
|||
# "text": text, |
|||
# "acc": output, |
|||
# }) |
|||
|
|||
output = torch.sigmoid(result[0]).tolist() |
|||
# print(output) |
|||
|
|||
# if output[0][0] > 0.60: |
|||
# predict_lable = 0 |
|||
# else: |
|||
# predict_lable = 1 |
|||
|
|||
max_index = max(enumerate(output[0]), key=lambda x: x[1])[0] |
|||
# print(max_index) # 输出最大值的下标 |
|||
jishu +=1 |
|||
data_new.append(json.dumps({ |
|||
"text": text, |
|||
"label": id_2_lable[max_index], |
|||
}, ensure_ascii=False)) |
|||
print(len(data_new)) |
|||
with open("data/data_title_content.jsonl", "a", encoding="utf-8") as f: |
|||
for i in data_new: |
|||
f.write(i) |
|||
f.write("\n") |
@ -0,0 +1,15 @@ |
|||
import pandas as pd |
|||
import random |
|||
|
|||
|
|||
data = pd.read_csv("data/train_data_weipu.csv").values.tolist() |
|||
random.shuffle(data) |
|||
random.shuffle(data) |
|||
|
|||
split = int(len(data)*0.5) |
|||
train_1 = data[:split] |
|||
train_2 = data[split:] |
|||
|
|||
pd.DataFrame(train_1, columns=["sentence", "label"]).to_csv("data/train_1_data_weipu.csv", index=False) |
|||
pd.DataFrame(train_2, columns=["sentence", "label"]).to_csv("data/train_2_data_weipu.csv", index=False) |
|||
|
@ -0,0 +1,39 @@ |
|||
import json |
|||
''' |
|||
{"text": "EU rejects German call to boycott British lamb.", "label": [[0, 2, "ORG"]]} |
|||
{"text": "Peter Blackburn", "label": [[0, 15, "PERSON"]]} |
|||
{"text": "President Obama", "label": [[10, 15, "PERSON"]]} |
|||
''' |
|||
with open("data/zc_4.jsonl", encoding="utf-8") as f: |
|||
data = f.readlines() |
|||
|
|||
data_new = [] |
|||
|
|||
for i in data: |
|||
data_dan_json = json.loads(i) |
|||
if len(data_dan_json["label"]) >1 or len(data_dan_json["label"]) == 0: |
|||
continue |
|||
text = data_dan_json["text"] |
|||
print(text) |
|||
print(data_dan_json) |
|||
|
|||
start = str(text).index("<\\Start>") |
|||
end = str(text).index("<\\End>") |
|||
|
|||
print(start) |
|||
print(end) |
|||
text = str(text).replace("<\\Start>", "").replace("<\\End>", "") |
|||
|
|||
label = [] |
|||
if data_dan_json['label'][0] != '正文': |
|||
label.append([start, end-8, data_dan_json['label'][0]]) |
|||
|
|||
data_new.append({ |
|||
"text": text, |
|||
"label": label |
|||
}) |
|||
|
|||
with open("data/zc_ner.jsonl", "a", encoding="utf-8") as f: |
|||
for i in data_new: |
|||
f.write(json.dumps(i, ensure_ascii=False)) |
|||
f.write("\n") |
@ -0,0 +1,135 @@ |
|||
import os |
|||
os.environ["WANDB_DISABLED"] = "true" |
|||
os.environ["CUDA_VISIBLE_DEVICES"] = "2" |
|||
import logging |
|||
import random |
|||
import sys |
|||
import warnings |
|||
from dataclasses import dataclass, field |
|||
from typing import Optional |
|||
import json |
|||
import datasets |
|||
import evaluate |
|||
import numpy as np |
|||
from datasets import load_dataset |
|||
import torch |
|||
import transformers |
|||
from transformers import ( |
|||
AutoConfig, |
|||
AutoModelForSequenceClassification, |
|||
BertForSequenceClassification, |
|||
AutoTokenizer, |
|||
DataCollatorWithPadding, |
|||
EvalPrediction, |
|||
HfArgumentParser, |
|||
PretrainedConfig, |
|||
Trainer, |
|||
TrainingArguments, |
|||
default_data_collator, |
|||
set_seed, |
|||
) |
|||
from transformers.trainer_utils import get_last_checkpoint |
|||
from transformers.utils import check_min_version, send_example_telemetry |
|||
from transformers.utils.versions import require_version |
|||
|
|||
|
|||
|
|||
def load_model(config_path: str, model_path: str): |
|||
model = AutoModelForSequenceClassification.from_pretrained(model_path) |
|||
tokenizer = AutoTokenizer.from_pretrained(model_path) |
|||
return model, tokenizer |
|||
|
|||
|
|||
def seq_padding(tokenizer, X): |
|||
pad_id = tokenizer.convert_tokens_to_ids("[PAD]") |
|||
if len(X) <= 1: |
|||
return torch.tensor(X) |
|||
L = [len(x) for x in X] |
|||
ML = max(L) |
|||
X = torch.Tensor([x + [pad_id] * (ML - len(x)) if len(x) < ML else x for x in X]) |
|||
return X |
|||
|
|||
|
|||
if __name__ == "__main__": |
|||
model, tokenizer = load_model(config_path='chinese_bert_wwm_ext_pytorch/config.json', |
|||
model_path='aigc_check') |
|||
|
|||
# text = "(1)经病理学或细胞学确诊的肺癌患者;" |
|||
# |
|||
# sen = [text] |
|||
# result = tokenizer(sen, max_length=512, truncation=True) |
|||
# print(result) |
|||
# |
|||
# input_ids = result['input_ids'] |
|||
# token_type_ids = result['token_type_ids'] |
|||
# |
|||
# input_ids = seq_padding(tokenizer, input_ids) |
|||
# token_type_ids = seq_padding(tokenizer, token_type_ids) |
|||
# |
|||
# |
|||
# result = model(input_ids=input_ids,token_type_ids=token_type_ids) # 这里不需要labels |
|||
# output = torch.sigmoid(result[0][0]).tolist() |
|||
# # result_ = result[0][0] |
|||
# print(output) |
|||
|
|||
model.to("cuda") |
|||
with open("data/paperred_aigc_cls.json", encoding='utf8') as f: |
|||
data = json.loads(f.read()) |
|||
|
|||
|
|||
data_new = [] |
|||
|
|||
zong = 0 |
|||
rel = 0 |
|||
jishu = 0 |
|||
|
|||
for index, i in enumerate(data[:10000]): |
|||
print(zong) |
|||
zong += 1 |
|||
text1 = i["input"] |
|||
text2 = i["output"] |
|||
|
|||
sen = [text1, text2] |
|||
result = tokenizer(sen, max_length=512, truncation=True) |
|||
|
|||
input_ids = result['input_ids'] |
|||
token_type_ids = result['token_type_ids'] |
|||
|
|||
input_ids = seq_padding(tokenizer, input_ids) |
|||
token_type_ids = seq_padding(tokenizer, token_type_ids) |
|||
|
|||
input_ids = input_ids.long() |
|||
token_type_ids = token_type_ids.long() |
|||
|
|||
batch_masks = input_ids.gt(0).to("cuda") |
|||
input_ids, token_type_ids = input_ids.to("cuda"), token_type_ids.to("cuda") |
|||
result = model(input_ids=input_ids,token_type_ids=token_type_ids, attention_mask=batch_masks) # 这里不需要labels |
|||
# output = torch.sigmoid(result[0][0]).tolist() |
|||
# # result_ = result[0][0] |
|||
# if output[1] > 0.5: |
|||
# rel += 1 |
|||
# |
|||
# data_new.append({ |
|||
# "index": index, |
|||
# "text": text, |
|||
# "acc": output, |
|||
# }) |
|||
|
|||
output = torch.sigmoid(result[0]).tolist() |
|||
if output[0][1] > 0.5 and output[1][1] < 0.5: |
|||
jishu +=1 |
|||
data_new.append({ |
|||
"index": index, |
|||
"text1": text1, |
|||
"text2": text2, |
|||
"acc": [output[0][1], output[1][1]], |
|||
}) |
|||
|
|||
print(jishu) |
|||
data_dict = { |
|||
# "acc" : rel/zong, |
|||
"data": data_new |
|||
} |
|||
|
|||
with open("data/paperred_aigc_acc3.json", "w", encoding='utf8') as f: |
|||
json.dump(data_dict, f, ensure_ascii=False, indent=4) |
@ -0,0 +1,108 @@ |
|||
import tensorflow as tf |
|||
import numpy as np |
|||
import os |
|||
|
|||
conll2003_path = "D:/ml/conll2003" |
|||
|
|||
|
|||
def load_file(path="/train.txt"): |
|||
# Load the dataset |
|||
train_sentences = [] |
|||
train_labels = [] |
|||
with open(conll2003_path + path) as f: |
|||
sentence = [] |
|||
labels = [] |
|||
for line in f: |
|||
line = line.strip() |
|||
if line: |
|||
word, pos, chunk, label = line.split() |
|||
sentence.append(word) |
|||
labels.append(label) |
|||
else: |
|||
train_sentences.append(sentence) |
|||
train_labels.append(labels) |
|||
sentence = [] |
|||
labels = [] |
|||
|
|||
return train_sentences, train_labels |
|||
|
|||
|
|||
return X, y |
|||
|
|||
|
|||
def get_dataset(): |
|||
# Load the dataset |
|||
train_sentences, train_labels = load_file("/train.txt") |
|||
valid_sentences, valid_labels = load_file("/valid.txt") |
|||
test_sentences, test_labels = load_file("/test.txt") |
|||
# Create vocabulary and tag dictionaries |
|||
all_sentencses = np.concatenate([train_sentences, valid_sentences, test_sentences]) |
|||
all_labels = np.concatenate([train_labels, valid_labels, test_labels]) |
|||
vocab = set() |
|||
tags = set() |
|||
for sentence in all_sentencses: |
|||
for word in sentence: |
|||
vocab.add(word.lower()) |
|||
for labels in all_labels: |
|||
for label in labels: |
|||
tags.add(label) |
|||
|
|||
word2idx = {w: i + 1 for i, w in enumerate(vocab)} |
|||
tag2idx = {t: i for i, t in enumerate(tags)} |
|||
|
|||
save_dict(word2idx, os.path.join('datasetpath', 'word2idx.json')) |
|||
save_dict(tag2idx, os.path.join('datasetpath', 'idx2Label.json')) |
|||
|
|||
num_words = len(word2idx) + 1 |
|||
num_tags = len(tag2idx) |
|||
|
|||
train_X, train_y = preproces(word2idx, tag2idx, num_tags, train_sentences, train_labels); |
|||
valid_X, valid_y = preproces(word2idx, tag2idx, num_tags, valid_sentences, valid_labels); |
|||
test_X, test_y = preproces(word2idx, tag2idx, num_tags, test_sentences, test_labels); |
|||
|
|||
np.savez(os.path.join('datasetpath', 'dataset.npz'), train_X=train_X, train_y=train_y, valid_X=valid_X, |
|||
valid_y=valid_y, test_X=test_X, test_y=test_y) |
|||
return train_X, train_y, valid_X, valid_y, test_X, test_y |
|||
|
|||
|
|||
def load_dataset(): |
|||
dataset = np.load(os.path.join('datasetpath', 'dataset.npz')) |
|||
train_X = dataset['train_X'] |
|||
train_y = dataset['train_y'] |
|||
valid_X = dataset['valid_X'] |
|||
valid_y = dataset['valid_y'] |
|||
test_X = dataset['test_X'] |
|||
test_y = dataset['test_y'] |
|||
return train_X, train_y, valid_X, valid_y, test_X, test_y |
|||
|
|||
|
|||
max_len = 64 |
|||
|
|||
|
|||
def save_dict(dict, file_path): |
|||
import json |
|||
# Saving the dictionary to a file |
|||
with open(file_path, 'w') as f: |
|||
json.dump(dict, f) |
|||
|
|||
|
|||
def load_dict(path_file): |
|||
import json |
|||
|
|||
# Loading the dictionary from the file |
|||
with open(path_file, 'r') as f: |
|||
loaded_dict = json.load(f) |
|||
return loaded_dict; |
|||
|
|||
print(loaded_dict) # Output: {'key1': 'value1', 'key2': 'value2'} |
|||
|
|||
|
|||
if __name__ == '__main__': |
|||
get_dataset() |
|||
train_X, train_y, valid_X, valid_y, test_X, test_y = load_dataset() |
|||
|
|||
print(len(train_X)) |
|||
print(len(train_y)) |
|||
|
|||
print(np.array(train_X).shape) |
|||
print(np.array(train_y).shape) |
@ -0,0 +1,22 @@ |
|||
from transformers import ( |
|||
AutoConfig, |
|||
AutoModelForTokenClassification, |
|||
AutoTokenizer, |
|||
DataCollatorForTokenClassification, |
|||
HfArgumentParser, |
|||
PretrainedConfig, |
|||
PreTrainedTokenizerFast, |
|||
Trainer, |
|||
TrainingArguments, |
|||
set_seed, |
|||
BertTokenizer, |
|||
BertTokenizerFast, |
|||
BertModel |
|||
) |
|||
|
|||
tokenizer = BertTokenizerFast.from_pretrained( |
|||
"/home/majiahui/project/models-llm/chinese-bigbird-wwm-base-4096", |
|||
use_fast=True, |
|||
revision="main", |
|||
trust_remote_code=False, |
|||
) |
@ -0,0 +1,132 @@ |
|||
import os |
|||
os.environ["WANDB_DISABLED"] = "true" |
|||
os.environ["CUDA_VISIBLE_DEVICES"] = "1" |
|||
import logging |
|||
import random |
|||
import sys |
|||
import warnings |
|||
from dataclasses import dataclass, field |
|||
from typing import Optional |
|||
import json |
|||
import datasets |
|||
import evaluate |
|||
import numpy as np |
|||
from datasets import load_dataset |
|||
import torch |
|||
import transformers |
|||
from transformers import ( |
|||
AutoConfig, |
|||
AutoModelForSequenceClassification, |
|||
BertForSequenceClassification, |
|||
AutoTokenizer, |
|||
DataCollatorWithPadding, |
|||
EvalPrediction, |
|||
HfArgumentParser, |
|||
PretrainedConfig, |
|||
Trainer, |
|||
TrainingArguments, |
|||
default_data_collator, |
|||
set_seed, |
|||
) |
|||
from transformers.trainer_utils import get_last_checkpoint |
|||
from transformers.utils import check_min_version, send_example_telemetry |
|||
from transformers.utils.versions import require_version |
|||
import pandas as pd |
|||
from tqdm import tqdm |
|||
|
|||
|
|||
def load_model(config_path: str, model_path: str): |
|||
model = AutoModelForSequenceClassification.from_pretrained(model_path) |
|||
tokenizer = AutoTokenizer.from_pretrained(model_path) |
|||
return model, tokenizer |
|||
|
|||
|
|||
def seq_padding(tokenizer, X): |
|||
pad_id = tokenizer.convert_tokens_to_ids("[PAD]") |
|||
if len(X) <= 1: |
|||
return torch.tensor(X) |
|||
L = [len(x) for x in X] |
|||
ML = max(L) |
|||
X = torch.Tensor([x + [pad_id] * (ML - len(x)) if len(x) < ML else x for x in X]) |
|||
return X |
|||
|
|||
|
|||
if __name__ == "__main__": |
|||
model, tokenizer = load_model(config_path='chinese_bert_wwm_ext_pytorch/config.json', |
|||
model_path='aigc_check_6') |
|||
|
|||
# text = "(1)经病理学或细胞学确诊的肺癌患者;" |
|||
# |
|||
# sen = [text] |
|||
# result = tokenizer(sen, max_length=512, truncation=True) |
|||
# print(result) |
|||
# |
|||
# input_ids = result['input_ids'] |
|||
# token_type_ids = result['token_type_ids'] |
|||
# |
|||
# input_ids = seq_padding(tokenizer, input_ids) |
|||
# token_type_ids = seq_padding(tokenizer, token_type_ids) |
|||
# |
|||
# |
|||
# result = model(input_ids=input_ids,token_type_ids=token_type_ids) # 这里不需要labels |
|||
# output = torch.sigmoid(result[0][0]).tolist() |
|||
# # result_ = result[0][0] |
|||
# print(output) |
|||
|
|||
model.to("cuda") |
|||
data_list = pd.read_csv("data/train_1_data_weipu.csv").values.tolist() |
|||
|
|||
data_new = [] |
|||
|
|||
zong = 0 |
|||
rel = 0 |
|||
jishu = 0 |
|||
|
|||
for i in tqdm(data_list): |
|||
# print(zong) |
|||
zong += 1 |
|||
text = i[0] |
|||
lable = i[1] |
|||
|
|||
result = tokenizer([text], max_length=512, truncation=True) |
|||
|
|||
input_ids = result['input_ids'] |
|||
token_type_ids = result['token_type_ids'] |
|||
# print(input_ids) |
|||
# print(text) |
|||
# print(lable) |
|||
input_ids = seq_padding(tokenizer, input_ids) |
|||
token_type_ids = seq_padding(tokenizer, token_type_ids) |
|||
|
|||
input_ids = input_ids.long() |
|||
token_type_ids = token_type_ids.long() |
|||
|
|||
batch_masks = input_ids.gt(0).to("cuda") |
|||
input_ids, token_type_ids = input_ids.to("cuda"), token_type_ids.to("cuda") |
|||
result = model(input_ids=input_ids,token_type_ids=token_type_ids, attention_mask=batch_masks) # 这里不需要labels |
|||
# output = torch.sigmoid(result[0][0]).tolist() |
|||
# # result_ = result[0][0] |
|||
# if output[1] > 0.5: |
|||
# rel += 1 |
|||
# |
|||
# data_new.append({ |
|||
# "index": index, |
|||
# "text": text, |
|||
# "acc": output, |
|||
# }) |
|||
|
|||
output = torch.sigmoid(result[0]).tolist() |
|||
# print(output) |
|||
|
|||
if output[0][0] > 0.50: |
|||
predict_lable = 0 |
|||
else: |
|||
predict_lable = 1 |
|||
jishu +=1 |
|||
|
|||
if predict_lable == lable: |
|||
data_new.append([ |
|||
text, |
|||
lable |
|||
]) |
|||
pd.DataFrame(data_new, columns=["sentence", "label"]).to_csv("data/train_2_2_data_weipu.csv.csv", index=False) |
@ -0,0 +1,14 @@ |
|||
import pandas as pd |
|||
|
|||
|
|||
data = pd.read_csv("data/dev_data_weipu.csv", encoding="utf-8").values.tolist() |
|||
|
|||
data_new = "" |
|||
for i in data[:100]: |
|||
data_new += i[0] |
|||
data_new += "\n" |
|||
|
|||
print(data_new) |
|||
|
|||
with open("data/ceshi_aigc_weipu.txt", "w", encoding="utf-8") as f: |
|||
f.write(data_new) |
@ -0,0 +1,2 @@ |
|||
a = 16+ 16 + 16 + 7+ 7 + 7+ 11 |
|||
print(a) |
@ -0,0 +1,33 @@ |
|||
# 定义目标表名 |
|||
import time |
|||
|
|||
target_tables = [ |
|||
"spider_latest_journal_paper_list", |
|||
"spider_latest_qikan_column_list", |
|||
"spider_latest_qikan_list" |
|||
] |
|||
|
|||
# 输出文件保存路径 |
|||
output_file = "found_tables.sql" |
|||
|
|||
# 初始化变量 |
|||
found_lines = [] |
|||
|
|||
|
|||
# 逐行读取文件 |
|||
with open("/home/majiahui/project/mysql_file_zw_paper/fabiao.sql", "r", encoding="utf-8") as file: |
|||
for line_number, line in enumerate(file, start=1): |
|||
if line_number%10000 == 0: |
|||
print("==============================================") |
|||
print(line_number) |
|||
print(time.time()) |
|||
for table in target_tables: |
|||
if table in line: |
|||
found_lines.append(f"Line {line_number}: {line.strip()}") |
|||
break |
|||
|
|||
# 保存匹配结果到文件 |
|||
with open(output_file, "w", encoding="utf-8") as outfile: |
|||
outfile.write("\n".join(found_lines)) |
|||
|
|||
print(f"搜索完成,共找到 {len(found_lines)} 行,结果已保存到 {output_file}") |
@ -0,0 +1,6 @@ |
|||
with open('found_tables.sql', 'r') as file: |
|||
for i, line in enumerate(file): |
|||
if i < 7: |
|||
print(line.strip()) |
|||
else: |
|||
break |
Loading…
Reference in new issue