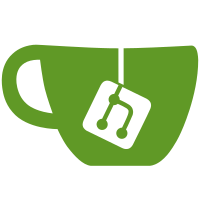
commit
fcb93c6326
9 changed files with 574 additions and 0 deletions
@ -0,0 +1,188 @@ |
|||
import os |
|||
os.environ["CUDA_VISIBLE_DEVICES"] = "0" |
|||
from flask import Flask, jsonify |
|||
from flask import request |
|||
import numpy as np |
|||
import faiss |
|||
import json |
|||
import requests |
|||
import socket |
|||
from sentence_transformers import SentenceTransformer |
|||
|
|||
|
|||
with open("data/lable/id2lable.json", encoding="utf-8") as f: |
|||
id2lable = json.loads(f.read()) |
|||
|
|||
with open("data/lable/lable2id.json", encoding="utf-8") as f: |
|||
lable2id = json.loads(f.read()) |
|||
|
|||
with open("data/discipline_types.json") as f: |
|||
lable_discipline_types = json.loads(f.read()) |
|||
|
|||
|
|||
app = Flask(__name__) |
|||
app.config["JSON_AS_ASCII"] = False |
|||
|
|||
d = 768 # dimension |
|||
model = SentenceTransformer('Dmeta-embedding-zh') |
|||
|
|||
def get_host_ip(): |
|||
""" |
|||
查询本机ip地址 |
|||
:return: ip |
|||
""" |
|||
try: |
|||
s = socket.socket(socket.AF_INET, socket.SOCK_DGRAM) |
|||
s.connect(('8.8.8.8', 80)) |
|||
ip = s.getsockname()[0] |
|||
finally: |
|||
s.close() |
|||
|
|||
return ip |
|||
|
|||
# url = "http://{}:50003/roformer".format(str(get_host_ip())) |
|||
url = "http://{}:50003/roformer".format("192.168.31.149") |
|||
|
|||
def dialog_line_parse(url, text): |
|||
""" |
|||
将数据输入模型进行分析并输出结果 |
|||
:param url: 模型url |
|||
:param text: 进入模型的数据 |
|||
:return: 模型返回结果 |
|||
""" |
|||
|
|||
response = requests.post( |
|||
url, |
|||
json=text, |
|||
timeout=1000 |
|||
) |
|||
if response.status_code == 200: |
|||
return response.json() |
|||
else: |
|||
# logger.error( |
|||
# "【{}】 Failed to get a proper response from remote " |
|||
# "server. Status Code: {}. Response: {}" |
|||
# "".format(url, response.status_code, response.text) |
|||
# ) |
|||
print("【{}】 Failed to get a proper response from remote " |
|||
"server. Status Code: {}. Response: {}" |
|||
"".format(url, response.status_code, response.text)) |
|||
print(text) |
|||
return [] |
|||
|
|||
def ulit_recall_paper(reference_list): |
|||
''' |
|||
对返回的十篇文章路径读取并解析 |
|||
:param recall_data_list_path: |
|||
:return data: list [[sentence, filename],[sentence, filename],[sentence, filename]] |
|||
''' |
|||
|
|||
# data = [] |
|||
# for path in recall_data_list_path: |
|||
# filename = path.split("/")[-1] |
|||
# with open(path, encoding="gbk") as f: |
|||
# text = f.read() |
|||
# text_list = text.split("\n") |
|||
# for sentence in text_list: |
|||
# if sentence != "": |
|||
# data.append([sentence, filename]) |
|||
# return data |
|||
|
|||
# recall_data_list |
|||
# 作者 论文名称 论文类别 论文来源 论文年份 摘要 |
|||
# "[1]赵璐.基于旅游资源开发下的新农村景观营建研究[D].西安建筑科技大学,2014." |
|||
data = [] |
|||
for data_one in reference_list: |
|||
paper = ".".join([ |
|||
",".join([i for i in data_one[0].split(";") if i != ""]), |
|||
data_one[1] + "[J]", |
|||
",".join([ |
|||
data_one[3], str(data_one[4]) + "." |
|||
]) |
|||
]) |
|||
|
|||
data.append(paper) |
|||
|
|||
return data |
|||
|
|||
|
|||
def main(title, abstract, nums): |
|||
data = { |
|||
"title": title, |
|||
"abst_zh": abstract, |
|||
"content": "" |
|||
} |
|||
# { |
|||
# "label_num": [ |
|||
# 117, |
|||
# 143 |
|||
# ] |
|||
# } |
|||
result = dialog_line_parse(url, data) |
|||
|
|||
# print(result['label_num'][0]) |
|||
# print(id2lable[result['label_num'][0]]) |
|||
subject_pinyin = lable_discipline_types[id2lable[str(result['label_num'][0])]] |
|||
|
|||
# with open(f"data/prompt/{subject_pinyin}.npy") as : |
|||
# zidonghua = np.load('data/prompt/{subject_pinyin}.npy') |
|||
|
|||
data_subject = np.load(f"data/prompt_qikan/{subject_pinyin}.npy") |
|||
|
|||
index = faiss.read_index(f'data/prompt_qikan_ivf/{subject_pinyin}.ivf') |
|||
|
|||
with open(f"data/data_info_qikan/{subject_pinyin}.json") as f: |
|||
data_info = json.loads(f.read()) |
|||
|
|||
index.add(data_subject) |
|||
# index.nprobe = 2 # default nprobe is 1, try a few more |
|||
k = nums |
|||
prompt = "标题:“{}”,摘要:“{}”".format(title, abstract) |
|||
embs = model.encode([prompt], normalize_embeddings=True) |
|||
|
|||
D, I = index.search(embs, int(k)) |
|||
print(I) |
|||
|
|||
reference_list = [] |
|||
abstract_list = [] |
|||
for i in I[0]: |
|||
reference_list.append(data_info[i]) |
|||
abstract_list.append(data_info[i][5]) |
|||
|
|||
return "200", ulit_recall_paper(reference_list), abstract_list |
|||
|
|||
|
|||
@app.route("/", methods=["POST"]) |
|||
def handle_query(): |
|||
# try: |
|||
title = request.form.get("title") |
|||
abstract = request.form.get('abstract') |
|||
nums = request.form.get('nums') |
|||
|
|||
# content = ulit_request_file(file) |
|||
|
|||
status_code, reference, abstract_list = main(title, abstract, nums) |
|||
|
|||
if status_code == "400": |
|||
return_text = {"resilt": "", "probabilities": None, "status_code": 400} |
|||
else: |
|||
reference_list = reference |
|||
print(reference_list) |
|||
reference = [f"[{str(i+1)}]" + reference_list[i] for i in range(len(reference_list))] |
|||
if status_code == "200": |
|||
return_text = { |
|||
"resilt": { |
|||
"reference": reference, |
|||
"abstract": abstract_list |
|||
}, |
|||
"probabilities": None, |
|||
"status_code": 200 |
|||
} |
|||
else: |
|||
return_text = {"resilt": "", "probabilities": None, "status_code": 400} |
|||
# except: |
|||
# return_text = {"resilt": "", "probabilities": None, "status_code": 400} |
|||
return jsonify(return_text) # 返回结果 |
|||
|
|||
if __name__ == "__main__": |
|||
app.run(host="0.0.0.0", port=17001, threaded=True) |
@ -0,0 +1,33 @@ |
|||
import pymysql |
|||
import json |
|||
|
|||
# 建立数据库连接 |
|||
connection = pymysql.connect( |
|||
host='rm-bp11ky2z5f34d2949fo.mysql.rds.aliyuncs.com', |
|||
user='fabiao_r', |
|||
password='f5u1w8nfb3b', |
|||
database='fabiao', |
|||
cursorclass=pymysql.cursors.DictCursor # 返回字典形式的结果,方便操作 |
|||
) |
|||
|
|||
try: |
|||
with connection.cursor() as cursor: |
|||
# 执行查询 |
|||
sql = "SELECT * FROM spider_latest_journal_paper_list" |
|||
cursor.execute(sql) |
|||
|
|||
# 获取查询结果 |
|||
result = cursor.fetchall() |
|||
print(len(result)) |
|||
|
|||
# 处理结果 |
|||
|
|||
# for row in result: |
|||
# print(row) |
|||
|
|||
with open("data/doctor_2018_2021.json", "w", encoding="utf-8") as f: |
|||
f.write(json.dumps(result, indent=2, ensure_ascii=False)) |
|||
|
|||
finally: |
|||
# 关闭连接 |
|||
connection.close() |
@ -0,0 +1,66 @@ |
|||
import json |
|||
|
|||
# json.load() |
|||
|
|||
# with open("t_xuewei_cnki_spider.csv", encoding="utf-8") as f: |
|||
# a = f.read() |
|||
# print(a) |
|||
|
|||
import pandas as pd |
|||
|
|||
filename = 'data/spider_latest_journal_paper_list.csv' |
|||
chunksize = 10000 # 指定每次读取的行数,可以根据需要调整 |
|||
|
|||
df_list = [] |
|||
# 使用 chunksize 参数迭代读取 CSV 文件 |
|||
for chunk in pd.read_csv(filename, chunksize=chunksize): |
|||
# 作者 论文名称 论文类别 论文来源 论文年份 摘要 |
|||
|
|||
# 对每个 chunk 进行处理 |
|||
|
|||
# print(chunk.columns) |
|||
# 9 / 0 |
|||
df_list_dan = chunk.values.tolist() |
|||
# print(df_list[0]) |
|||
for i in range(len(df_list_dan)): |
|||
df_list.append({ |
|||
'author': df_list_dan[i][2], |
|||
'title': df_list_dan[i][1], |
|||
'special_topic': df_list_dan[i][7], |
|||
'qikan_name': df_list_dan[i][3], |
|||
'year': df_list_dan[i][4], |
|||
'abstract': df_list_dan[i][10], |
|||
}) |
|||
|
|||
# data = [] |
|||
# json_list = [ |
|||
# "/home/majiahui/project/爬取目录筛选/t_xuewei_detail_cnki.json", |
|||
# "/home/majiahui/project/爬取目录筛选/t_xuewei_detail_cnki2.json", |
|||
# "/home/majiahui/project/爬取目录筛选/t_xuewei_detail_cnki3.json", |
|||
# "/home/majiahui/project/爬取目录筛选/t_xuewei_detail_cnki6.json", |
|||
# "/home/majiahui/project/爬取目录筛选/t_xuewei_detail_cnki7.json", |
|||
# ] |
|||
# |
|||
# |
|||
# print("主库数据完成加载") |
|||
# for path in json_list: |
|||
# name, typr_file = path.split(".") |
|||
# name = name.split("/")[-1] |
|||
# a = json.load(open(path)) |
|||
# for i in a: |
|||
# autoid = "_".join([name, str(i['autoid'])]) |
|||
# if autoid in df_dict: |
|||
# data.append([i['f_title']] + df_dict[autoid]) |
|||
# print("path完成筛选") |
|||
# |
|||
# |
|||
with open("data/data_0416.json", "w") as f: |
|||
f.write(json.dumps(df_list, ensure_ascii=False, indent=2)) |
|||
|
|||
# |
|||
# with open("data.json", encoding="utf-8") as f: |
|||
# for i in f.readlines(): |
|||
# a = json.loads(i) |
|||
# |
|||
# |
|||
# print(a) |
@ -0,0 +1,80 @@ |
|||
import os |
|||
os.environ["CUDA_VISIBLE_DEVICES"] = "0" |
|||
import json |
|||
import numpy as np |
|||
from tqdm import tqdm |
|||
from sentence_transformers import SentenceTransformer |
|||
|
|||
model = SentenceTransformer('Dmeta-embedding-zh') |
|||
print(1) |
|||
with open("data/discipline_types.json", encoding="utf-8") as f: |
|||
lable_discipline_types = json.loads(f.read()) |
|||
|
|||
def erjimul_ulit(): |
|||
pass |
|||
|
|||
def shengcehng_array(data): |
|||
embs = model.encode(data, normalize_embeddings=True) |
|||
return embs |
|||
|
|||
|
|||
if __name__ == '__main__': |
|||
|
|||
# data = [] |
|||
with open("data/data_0416.json", encoding="utf-8") as f: |
|||
# for i in f.readlines(): |
|||
# a = json.loads(i) |
|||
# data.append(a) |
|||
data = json.loads(f.read()) |
|||
|
|||
print(len(data)) |
|||
|
|||
a = 0 |
|||
|
|||
a_ = 0 |
|||
data_info = {} # 作者 论文名称 论文类别 论文来源 论文年份 摘要 |
|||
data_prompt = {} |
|||
for data_dan in data: |
|||
if str(data_dan["special_topic"]) == "nan": |
|||
a_ += 1 |
|||
continue |
|||
|
|||
leibie_list = data_dan["special_topic"].split(";") |
|||
for leibie in leibie_list: |
|||
if leibie in lable_discipline_types: |
|||
if lable_discipline_types[leibie] not in data_prompt: |
|||
data_prompt[lable_discipline_types[leibie]] = ["标题:“{}”,摘要:“{}”".format(data_dan["title"], data_dan["abstract"])] |
|||
data_info[lable_discipline_types[leibie]] = [[data_dan["author"], data_dan["title"], data_dan["special_topic"], data_dan["qikan_name"], data_dan["year"], data_dan["abstract"], "期刊"]] |
|||
else: |
|||
data_prompt[lable_discipline_types[leibie]].append("标题:“{}”,摘要:“{}”".format(data_dan["title"], data_dan["abstract"])) |
|||
data_info[lable_discipline_types[leibie]].append([data_dan["author"], data_dan["title"], data_dan["special_topic"], data_dan["qikan_name"], data_dan["year"], data_dan["abstract"], "期刊"]) |
|||
a += 1 |
|||
|
|||
print(2) |
|||
strat = 0 |
|||
end = 10000 |
|||
print(len(data_prompt)) |
|||
for leibie in tqdm(data_prompt): |
|||
data_ndarray = np.empty((0, 768)) |
|||
print("len(data_prompt[leibie])", len(data_prompt[leibie])) |
|||
while True: |
|||
if end >= len(data_prompt[leibie]): |
|||
break |
|||
linshi_data = data_prompt[leibie][strat:end] |
|||
data_ndarray = np.concatenate((data_ndarray, shengcehng_array(linshi_data))) |
|||
print("data_ndarray.shape", data_ndarray.shape) |
|||
strat = end |
|||
end += 10000 |
|||
|
|||
linshi_data = data_prompt[leibie][strat:len(data_prompt[leibie])] |
|||
print("len(linshi_data)", len(linshi_data)) |
|||
data_ndarray = np.concatenate((data_ndarray, shengcehng_array(linshi_data))) |
|||
print("data_ndarray.shape", data_ndarray.shape) |
|||
np.save(f'data/prompt_qikan/{leibie}.npy', data_ndarray) |
|||
strat = 0 |
|||
end = 10000 |
|||
|
|||
for leibie in data_info: |
|||
print(len(data_info[leibie])) |
|||
with open(f"data/data_info_qikan/{leibie}.json", "w", encoding="utf-8") as f: |
|||
f.write(json.dumps(data_info[leibie], ensure_ascii=False, indent=2)) |
@ -0,0 +1,66 @@ |
|||
import json |
|||
|
|||
# json.load() |
|||
|
|||
# with open("t_xuewei_cnki_spider.csv", encoding="utf-8") as f: |
|||
# a = f.read() |
|||
# print(a) |
|||
|
|||
import pandas as pd |
|||
|
|||
filename = 'data/spider_latest_journal_paper_list.csv' |
|||
chunksize = 10000 # 指定每次读取的行数,可以根据需要调整 |
|||
|
|||
df_list = [] |
|||
# 使用 chunksize 参数迭代读取 CSV 文件 |
|||
for chunk in pd.read_csv(filename, chunksize=chunksize): |
|||
# 作者 论文名称 论文类别 论文来源 论文年份 摘要 |
|||
|
|||
# 对每个 chunk 进行处理 |
|||
|
|||
# print(chunk.columns) |
|||
# 9 / 0 |
|||
df_list_dan = chunk.values.tolist() |
|||
# print(df_list[0]) |
|||
for i in range(len(df_list_dan)): |
|||
df_list.append({ |
|||
'author': df_list_dan[i][2], |
|||
'title': df_list_dan[i][1], |
|||
'special_topic': df_list_dan[i][7], |
|||
'qikan_name': df_list_dan[i][3], |
|||
'year': df_list_dan[i][4], |
|||
'abstract': df_list_dan[i][10], |
|||
}) |
|||
|
|||
# data = [] |
|||
# json_list = [ |
|||
# "/home/majiahui/project/爬取目录筛选/t_xuewei_detail_cnki.json", |
|||
# "/home/majiahui/project/爬取目录筛选/t_xuewei_detail_cnki2.json", |
|||
# "/home/majiahui/project/爬取目录筛选/t_xuewei_detail_cnki3.json", |
|||
# "/home/majiahui/project/爬取目录筛选/t_xuewei_detail_cnki6.json", |
|||
# "/home/majiahui/project/爬取目录筛选/t_xuewei_detail_cnki7.json", |
|||
# ] |
|||
# |
|||
# |
|||
# print("主库数据完成加载") |
|||
# for path in json_list: |
|||
# name, typr_file = path.split(".") |
|||
# name = name.split("/")[-1] |
|||
# a = json.load(open(path)) |
|||
# for i in a: |
|||
# autoid = "_".join([name, str(i['autoid'])]) |
|||
# if autoid in df_dict: |
|||
# data.append([i['f_title']] + df_dict[autoid]) |
|||
# print("path完成筛选") |
|||
# |
|||
# |
|||
with open("data/data_0416.json", "w") as f: |
|||
f.write(json.dumps(df_list, ensure_ascii=False, indent=2)) |
|||
|
|||
# |
|||
# with open("data.json", encoding="utf-8") as f: |
|||
# for i in f.readlines(): |
|||
# a = json.loads(i) |
|||
# |
|||
# |
|||
# print(a) |
@ -0,0 +1,53 @@ |
|||
import os |
|||
os.environ["CUDA_VISIBLE_DEVICES"] = "0" |
|||
|
|||
import numpy as np |
|||
import faiss |
|||
import json |
|||
from sentence_transformers import SentenceTransformer |
|||
|
|||
d = 768 # dimension |
|||
zidonghua = np.load('zidonghua.npy') |
|||
model = SentenceTransformer('Dmeta-embedding-zh') |
|||
|
|||
data = [] |
|||
with open("data.json", encoding="utf-8") as f: |
|||
for i in f.readlines(): |
|||
a = json.loads(i) |
|||
data.append(a) |
|||
|
|||
mubiaoliebie = "自动化技术" |
|||
data_prompt = [] |
|||
for i in data: |
|||
if str(i[1]) == "nan": |
|||
continue |
|||
|
|||
leibie_list = i[1].split(";") |
|||
for leibie in leibie_list: |
|||
if leibie == mubiaoliebie: |
|||
data_prompt.append("标题:“{}”,摘要:“{}”".format(i[0], i[2])) |
|||
|
|||
|
|||
|
|||
# faiss.write_index(index, 'index.ivf') |
|||
index = faiss.read_index('zidonghua.ivf') |
|||
|
|||
index.add(zidonghua) # add may be a bit slower as well |
|||
# D, I = index.search(xq, k) # actual search |
|||
# print(I[-5:]) # neighbors of the 5 last queries |
|||
|
|||
|
|||
print("=======================================") |
|||
index.nprobe = 2 # default nprobe is 1, try a few more |
|||
k = 4 |
|||
biaoti = "工业机器人视觉导航系统的设计与实现" |
|||
zhaiyoa = "本研究致力于设计和实现工业机器人视觉导航系统,旨在提高工业生产中机器人的自主导航和定位能力。首先,通过综合考虑视觉传感器、定位算法和控制策略,设计了一种高效的机器人视觉导航系统框架。其次,利用深度学习技术对环境中的关键特征进行识别和定位,实现了机器人在复杂工作场景下的精确定位和路径规划。通过实验验证,本系统在提高机器人工作效率、减少人工干预以及降低操作误差等方面取得了显著的成果。因此,本研究为工业机器人在生产领域的应用提供了重要的技术支持,具有一定的实用和推广价值。" |
|||
|
|||
prompt = "标题:“{}”,摘要:“{}”".format(biaoti, zhaiyoa) |
|||
embs = model.encode([prompt], normalize_embeddings=True) |
|||
|
|||
D, I = index.search(embs, k) |
|||
print(I) |
|||
|
|||
for i in I[0]: |
|||
print(data_prompt[i]) |
@ -0,0 +1,23 @@ |
|||
import json |
|||
|
|||
with open("label_threshold.txt", encoding="utf-8") as f: |
|||
data = json.loads(f.read()) |
|||
|
|||
|
|||
id2lable = {} |
|||
lable2id = {} |
|||
for i in data: |
|||
if i not in lable2id: |
|||
lable2id[i] = data[i][0] |
|||
|
|||
for i in lable2id: |
|||
if lable2id[i] not in id2lable: |
|||
id2lable[lable2id[i]] = i |
|||
|
|||
|
|||
with open("data/lable/id2lable.json", "w", encoding="utf-8") as f: |
|||
f.write(json.dumps(id2lable, indent=2, ensure_ascii=False)) |
|||
|
|||
|
|||
with open("data/lable/lable2id.json", "w", encoding="utf-8") as f: |
|||
f.write(json.dumps(lable2id, indent=2, ensure_ascii=False)) |
@ -0,0 +1,31 @@ |
|||
import json |
|||
from pypinyin import pinyin, Style |
|||
import pandas as pd |
|||
|
|||
|
|||
def hanzi_to_pinyin(hanzi): |
|||
# 将汉字转换为拼音,Style.NORMAL表示以带音调的拼音形式输出 |
|||
pinyin_list = pinyin(hanzi, style=Style.NORMAL, heteronym=False) |
|||
print(pinyin_list) |
|||
# 将拼音列表连接成字符串 |
|||
pinyin_str = ''.join([i[0] for i in pinyin_list]) |
|||
return pinyin_str |
|||
|
|||
|
|||
if __name__ == '__main__': |
|||
df_list = pd.read_excel("论文种类分类表1.xls").values.tolist() |
|||
print(df_list) |
|||
|
|||
erji_dict = {} |
|||
|
|||
for i in range(len(df_list)): |
|||
if str(df_list[i][1]) == "nan": |
|||
continue |
|||
if df_list[i][1] not in erji_dict : |
|||
erji_dict[df_list[i][1]] = hanzi_to_pinyin(df_list[i][1]) |
|||
|
|||
print(erji_dict) |
|||
print(len(erji_dict)) |
|||
|
|||
with open("discipline_types.json", "w", encoding="utf-8") as f: |
|||
f.write(json.dumps(erji_dict, ensure_ascii=False, indent=2)) |
@ -0,0 +1,34 @@ |
|||
import numpy as np |
|||
import faiss |
|||
import json |
|||
import math |
|||
|
|||
|
|||
d = 768 # dimension |
|||
# nlist = 1000 #聚类的数目 |
|||
|
|||
|
|||
with open("data/discipline_types.json") as f: |
|||
lable_discipline_types = json.loads(f.read()) |
|||
|
|||
a = 0 |
|||
for leibie_zh in lable_discipline_types: |
|||
|
|||
xb = np.load(f'data/prompt_qikan/{lable_discipline_types[leibie_zh]}.npy') |
|||
|
|||
# nlist = math.floor((len(lable_discipline_types[leibie_zh]) ** 0.5)) # 聚类的数目 |
|||
# print(leibie_zh) |
|||
# print(len(lable_discipline_types[leibie_zh])) |
|||
# print(nlist) |
|||
|
|||
print(xb.shape) |
|||
nlist = math.floor((xb.shape[0] ** 0.5)) |
|||
a += xb.shape[0] |
|||
print(nlist) |
|||
quantizer = faiss.IndexFlatL2(d) |
|||
index = faiss.IndexIVFFlat(quantizer, d, nlist) |
|||
assert not index.is_trained |
|||
index.train(xb) # IndexIVFFlat是需要训练的,这边是学习聚类 |
|||
assert index.is_trained |
|||
faiss.write_index(index, f'data/prompt_qikan_ivf/{lable_discipline_types[leibie_zh]}.ivf') |
|||
print(a) |
Loading…
Reference in new issue