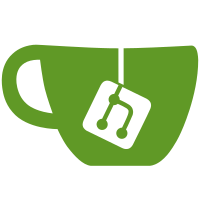
6 changed files with 193 additions and 117 deletions
@ -0,0 +1,32 @@ |
|||
# -*- coding: utf-8 -*- |
|||
|
|||
""" |
|||
@Time : 2023/3/27 10:23 |
|||
@Author : |
|||
@FileName: |
|||
@Software: |
|||
@Describe: |
|||
""" |
|||
import sys |
|||
import os |
|||
|
|||
pre_model_path = { |
|||
"simbert": { |
|||
"linux": "/home/zc-nlp-zyp/work_file/ssd_data/模型库/预训练模型集合/keras/chinese_roberta_wwm_ext_L-12_H-768_A-12", |
|||
"win32": r"E:\pycharm_workspace\premodel\keras\chinese_roberta_wwm_ext_L-12_H-768_A-12" |
|||
}, |
|||
} |
|||
|
|||
|
|||
class DropSimBertConfig: |
|||
def __init__(self): |
|||
self.sys_platform = sys.platform |
|||
self.premodel_path = pre_model_path["simbert"][self.sys_platform] |
|||
self.config_path = os.path.join(self.premodel_path, 'bert_config.json') |
|||
self.checkpoint_path = os.path.join(self.premodel_path, 'bert_model.ckpt') |
|||
|
|||
self.dict_path = './config/vocab_drop.txt' |
|||
self.savemodel_path = "./output_simbert_yy/best_simbertmodel_dropout_datasim_yinhao.weights" |
|||
|
|||
self.maxlen = 120 |
|||
self.cuda_id = "1" |
@ -0,0 +1,45 @@ |
|||
# -*- coding: utf-8 -*- |
|||
|
|||
""" |
|||
@Time : 2023/3/27 10:23 |
|||
@Author : |
|||
@FileName: |
|||
@Software: |
|||
@Describe: |
|||
""" |
|||
import sys |
|||
import os |
|||
|
|||
pre_model_path = { |
|||
"t5": { |
|||
"linux": "/home/zc-nlp-zyp/work_file/ssd_data/模型库/预训练模型集合/keras/mt5/mt5_base", |
|||
"win32": r"E:\pycharm_workspace\premodel\keras\mt5\mt5_base" |
|||
}, |
|||
|
|||
} |
|||
|
|||
|
|||
class DropT5Config: |
|||
def __init__(self): |
|||
self.sys_platform = sys.platform |
|||
self.premodel_path = pre_model_path["t5"][self.sys_platform] |
|||
self.config_path = os.path.join(self.premodel_path, 'mt5_base_config.json') |
|||
self.checkpoint_path = os.path.join(self.premodel_path, 'model.ckpt-1000000') |
|||
self.spm_path = os.path.join(self.premodel_path, 'sentencepiece_cn.model') |
|||
self.keep_tokens_path = os.path.join(self.premodel_path, 'sentencepiece_cn_keep_tokens.json') |
|||
self.savemodel_path = "./output_t5/best_model_t5_zong_sim_99.weights" |
|||
self.maxlen = 256 |
|||
self.cuda_id = "1" |
|||
|
|||
|
|||
class MultipleResultsDropT5Config: |
|||
def __init__(self): |
|||
self.sys_platform = sys.platform |
|||
self.premodel_path = pre_model_path["t5"][self.sys_platform] |
|||
self.config_path = os.path.join(self.premodel_path, 'mt5_base_config.json') |
|||
self.checkpoint_path = os.path.join(self.premodel_path, 'model.ckpt-1000000') |
|||
self.spm_path = os.path.join(self.premodel_path, 'sentencepiece_cn.model') |
|||
self.keep_tokens_path = os.path.join(self.premodel_path, 'sentencepiece_cn_keep_tokens.json') |
|||
self.savemodel_path = "./output_t5/best_model_t5_dropout_0_3.weights" |
|||
self.maxlen = 256 |
|||
self.cuda_id = "1" |
Loading…
Reference in new issue